Sumit Sanyal
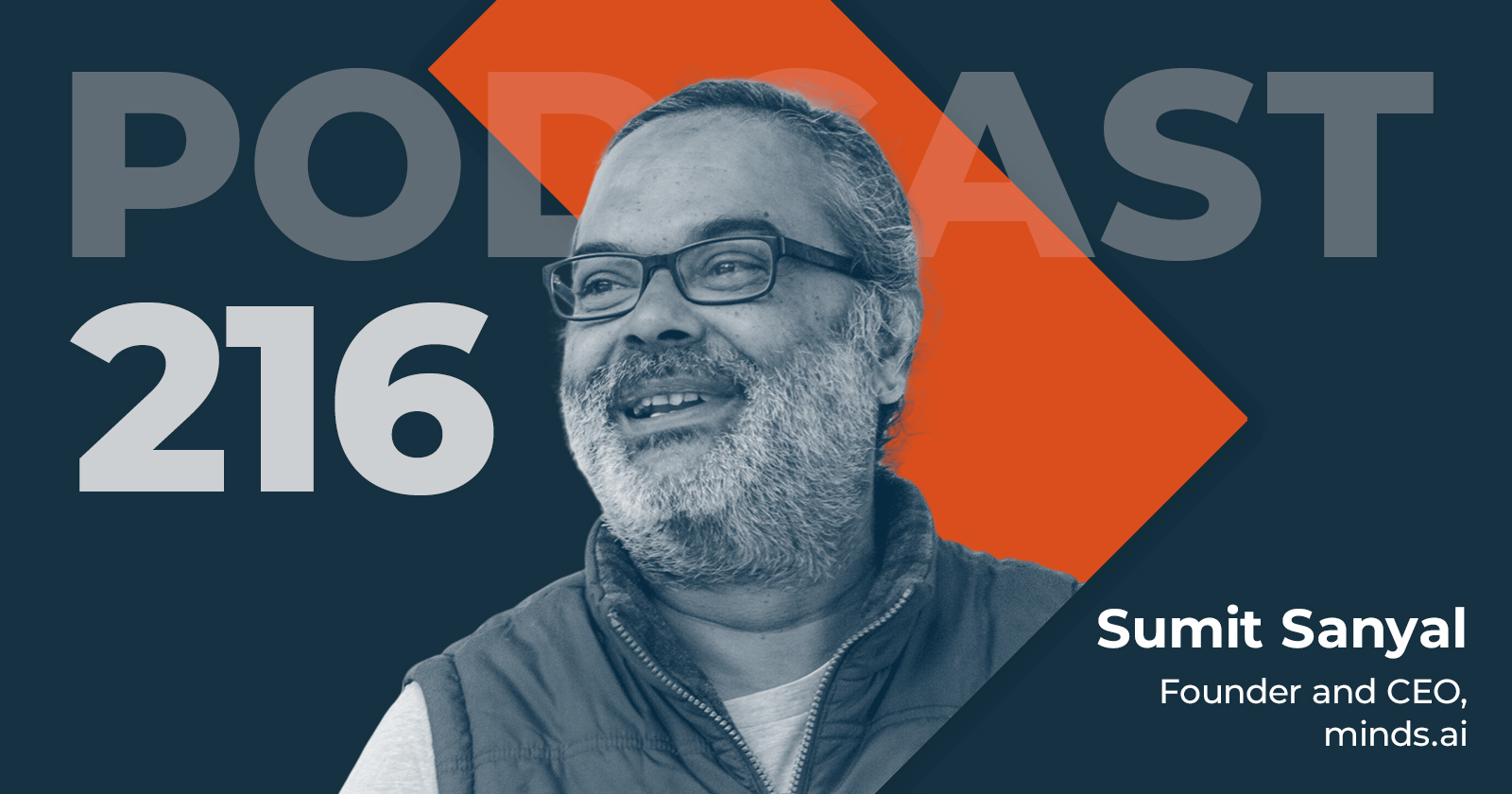
Ken: Good day, and welcome to episode 216 of our Momenta Digital Thread podcast series. Today, I'm pleased to host Sumit Sanyal, co-founder and CEO of minds.ai, a startup using artificial intelligence to optimize some of the most critical industries on the planet. Minds.ai is Momenta's most recent investment. Sumit is an experienced chief executive officer with a demonstrated history of success in the computer software industry. He has a proven track record in designing enterprise software, machine learning, embedded software, systems on a chip, and high-performance computing, all backed by a robust entrepreneurial skill set. Sumit is also a graduate of Indian Institute of Technology in Kanpur. Sumit, welcome to our Digital Thread podcast today.
[00:01:27]
Sumit: Good morning, Ken. Thanks for having me; both for the trust that Momenta has placed in minds.ai with your investment and, of course, for having me on your podcast. I'm looking forward to our conversation.
[00:01:44]
Ken: As well, and as I was hinting in our pre-conversation, you've come highly recommended, both as a speaker and a compelling entrepreneur. Of course, we've already invested, knowing that you can operate and scale a company, which we're also quite excited about. We call this the Digital Thread podcast, so we always ask the question up front: what would you consider to be your digital thread? In other words, the one or more thematic threads that define your digital industry journey.
[00:02:14]
Sumit: Indeed, and I love the theme of this podcast, digital threads. Also, I'm always curious to know the stories behind successful startup founders and the stories some of these we hear. I'm happy you're doing this. I've listened to a few on the series, and it's quite an exciting theme. About myself- it's pretty simple. I had a passion almost bordering on an obsession since I put my first PC together when I was young. That's what made computers work; I wanted to understand that. I remember, as a kid, taking apart the motherboard of the PC and looking at the black rectangular piece of hard plastic inside and staring at it and thinking, "What is the magic that goes inside this?" It's been a journey that motivated me to take electrical engineering as my engineering degree. The choice was between wanting to learn how to program computers or building computers. All along, I've taken the choice of- I want to learn how to build computers, and that led me to an electrical engineering degree. In terms of the macroeconomic trends- big picture trends, if I look back at my career, the first one was the telecom revolution. After I graduated and when I came to this country from India, the telecom revolution was very much in swing. I designed custom hardware for various telecom parts and base stations, etc. It was the telecom journey.
The next revolution was what I call the smartphone revolution. That was the big brand. I was also front and center designing various parts for the smartphone revolution. If I look back, one of the highlights of my career is designing Bluetooth and Wi-Fi chips, which was a Broadcom part. I was at Broadcom at that time, the architect for these chips- we were selling it to Apple because, of course, going into the very first iPhones. I wanted to know how computers work, getting into the innards of it, rather than going to general purpose computing because of the kind of global trends. I went into SOC design and application-specific computing. Of course, the next revolution I got hit by was the AI revolution. At that point, I went from the design of these computing devices to the manufacturing of these computing devices, which brings us to the story of minds.ai.
[00:05:01]
Ken: It's interesting. First of all, just a comment on your story on digital threads. VCs invest in people, first and foremost. People who have ideas, defensible ideas, profitable ideas, etc., but it's people at the center, right? I fully agree with you. Sometimes, the stories about the people and what made them successful are as interesting as what they've accomplished. That was, in essence, the origin story for the podcast. Now, it's interesting concerning your being in the right place at the right time and truly working across the ecosystem. I was intrigued. You've done enterprise software design, system on a chip, digital signal processing, and wireless audio applications. Of course, now you're running an AI company. To what do you attribute this engineering dexterity?
[00:05:54]
Sumit: Curiosity, right? Curiosity. I'm always learning. If I'm not learning at my current job, then I don't want to be doing it. I get bored. It's just an insatiable thirst to keep learning, and, as I said, the big picture trajectory is, indeed- first, the telecom revolution. That's where I was doing base station software- enterprise software, whatever it takes to solve the problem. The telecom part of my career was mostly on heavy iron data center enterprise-level solutions behind the base station, off the cellular network. But then, throughout my career, I graduated more and more towards the benefits of the network, which is graduated towards the handhelds. I did a bunch of hardware design and systems design for the mobile devices because that was the complete craze, and see, the other part of it is I'm surfing the wave. I'm humble enough to know that I'm just a part of this bigger kind of transition that's happening- the technology revolution that's happening. I surf the wave of the telecom wave, and by nature, I like to be on the bleeding edge of those waves. There was the telecom revolution, and then there was the smartphone revolution. I've been fortunate enough to identify these trends early now and jump onto them.
[00:07:36]
Ken: I noted your last engineering role before minds.ai described your work as designing a better system on a chip to do audio signal processing with a deep neural net. Was this the beginning of your journey with AI and, thus, minds.ai?
[00:07:52]
Sumit: Indeed, it was, Ken. Indeed. There is quite a story there. I was the systems architect, and we were building a chip designed to do speech recognition, echo cancellation, noise reduction, etc., very low power and latency applications, which were the need of the hour for cell phones. At that time, the state of the art in terms of speech recognition was stuck at about 80% of recognition activities. The average human being is about 99.5% accurate in the sense that if you and I are talking every 200 words, I will miss it, but in a clear enough channel. 99.5% is human recognition accuracy for an average language speaker. The machines were stuck at 80%, which was pathetic, and it had been stopped for decades. At Broadcom and at Intel and companies like this, I've been fortunate enough to work with some of the world's leading experts on speech recognition, echo cancellation using conventional signal processing means like FFT and filter design, and stuff like this and all of that accumulated knowledge. The state of the art worldwide was stuck at 80%. Over 30 years, they had managed to eke it up every year- maybe it would go up by half a percent or 1%. My job was to implement these state-of-the-art algorithms in Silicon so that we could sell them to the smartphone vendors.
I read this paper at that time from two kids at Google. I mean kids in the sense that they were young and had no prior background in signal processing and retrieval to work for a particular conference; they were able to move the state of the art from 80% to 85%. That was a very basic, straightforward application of neural nets. I read this paper, and I see the writing on the wall. This is going to change everything. I walked into my CEO's office and told him we needed to pivot and pivot hard. We needed to put these neural processing chips into our smartphones. The CEO said, "You have no idea what you're talking about." I quit. I quit right there in the CEO's office and walked out. The very next day, I tried to start my own deep-learning company. It's another example of being way too ahead of our times because it's finally- gosh. Ten years later, that same neural network processing software is going into smartphones and, of course, the latest Pixel phone; Google is touting it false that it's got a neural network processing chip in there, etc. That's what I had pitched back to my CEO, and I saw the writing on the wall. We saw this happen, the AI revolution, one field after another. First, it was speech, and then it was image recognition. Of course, the image recognition stories are legendary, and their efforts devastated records in one field after the other. I saw that coming and have been surfing that wave since.
[00:11:19]
Ken: Tell us about the origin story of minds.ai, which you founded in 2014.
[00:11:25]
Sumit: We discussed the trigger event, a paper for speech recognition using deep neural nets. I saw the potential for these neural networks to revolutionize both the fields that- at that point, I considered myself an expert in. One was telecom and building telecom hardware, and the other was signal processing. That was the motivation to start the company, and it was a very sharp focus on deep neural nets. Machine learning and AI are terms that are often abused, way overloaded, and have ambiguous meanings. I like to focus on deep learning, which specifically refers to this class of algorithms- a neural network that stacks deep layers of neural networks, which does all its magic. That was indeed the genesis of the company. Then I looked around- I did not have any prior expertise in deep learning, so I looked around, and I was able to contact Tijmen Tieleman, who was, at that point, just finishing his Ph.D. under Geoffrey Hinton, in Geoffrey Hinton's lab in Toronto. Tijmen was looking for something to do; I went and pitched my startup idea to him and got him excited. That was it. Tijmen and I co-founded the company. At that point, we were building bespoke neural network solutions for enterprises.
[00:13:07]
Ken: Your key product, Maestro, applies supervised learning, reinforcement learning, and generative AI to key pain points in the semiconductor industry. What have been some of your notable use cases and wins there?
[00:13:20]
Sumit: Yes, the semiconductor industry is one of the most complex manufacturing environments on the planet. To give you a scale of things- the capital cost to build out and fill out a typical semiconductor manufacturing factory, which, in the jargon, they're called 'fabs'- is $20 billion. These are some of the planet's most sophisticated and complex manufacturing environments. It sounds like a boring topic, but to motivate the interest of your audience, we have to realize that these factories are putting out these semiconductor chips, ubiquitous in our modern lives. At this point, semiconductors are so basic to everything that we do as humanity that it's almost at the level of basic services like water and electricity, to the point that the US government identified semiconductor supply chain security as a homeland security threat as it affects everything that underpins modern civilization. These factories are churning out these bits of semiconductor power circuits, which are powering all of what we do as humanity, from our washing machines to our smartphones to our Cloud and every technology revolution we are talking about. It's underpinned by this $600 billion semiconductor industry. The manufacturing of these semiconductors is the part that we are focusing on, and we are bringing the AI revolution to this industry. One of the specific use cases we would like to discuss is the semiconductor manufacturing process- I'll use an analogy: a very busy airport with lots of flights, landing, and taking off. You can see that there are shared resources, which is the runway. You can see that there are mission-critical timing constraints involved, and you can see that there are hundreds or even thousands of planes that have to be distributed across hundreds of runways. That motivates the complexity of managing the wafer and production flow in one of these semiconductor manufacturing factories. One of the use cases we have had tremendous success in is applying the reinforcement learning technique to scheduling the movement of the raw material, which is the wafers, through the factories so that a finished product can come out at the other end.
The other point is that these products are complex; each individual product is hundreds of layers deep, and each layer requires a dozen or so steps. Each of those steps is the very familiar kind of photographic development technique where you put a layer, expose it with some light that changes the chemistry in some parts, and then etch it. You dry it, and you've got a photo. This step you have to repeat for every layer, and there are hundreds of layers, and that's just for one kind of semiconductor part at any given time in these modern factories. You could have 20, 30, 40, or even hundreds of such parts, each of which has its own recipe of manufacturing flowing through this complex flow. This is too complex and too high-dimensional an optimization problem for the human brain or even classical algorithms. We have taken reinforcement learning, which your listeners might be familiar with; it's the same algorithm used to win the chess-playing and the goal-playing machines. Reinforcement learning is the same kind of algorithm that DeepMind is famous for and has been used very effectively in things like AlphaGo and Alpha Fold, which is protein folding. DeepMind pioneered the commercialization- and application of reinforcement learning. We are one of the few outfits in the world outside of DeepMind that has successfully commercialized the applications of deep learning to the control and the organization and optimization day-by-day, hour-by-hour, and minute-by-minute optimization of the semiconductor manufacturing plants so that we can dramatically increase their productivity, reduce their waste, reduce the manufacturing cycle time of these critical and very important semiconductor components.
[00:18:23]
Ken: It's interesting to see the DNA; your background in semiconductors helps you appreciate the manufacturing processes and the complexity. Then, as you say, your curiosity led you to AI and particularly adopting DeepMind's approach to the manufacturing process. It all makes sense how you got together. If that were the value proposition, it would be interesting but not 100% compelling. What you guys have done that makes it compelling is that you're using AI to optimize some of the most important industries on the planet with zero downtime and zero disruption in established workflows. For our listeners, what is it that you consider to be this zero-downtime value proposition? How does that show up in practice?
[00:19:14]
Sumit: This 'zero-downtime' phrase is bandied about willy-nilly in many industries. But we must understand that for this kind of semiconductor factory, zero downtime is very, very real. I almost like to think of the fab as an organism with a birth, a lifetime, and a death. Like biological organisms, we live out our 80, 100-year lifespans and don't shut down. There is no downtime. Downtime means your heart stops, your brain goes, and you're dead. The semiconductor fab has a lifetime. Another analogy to the human body or a biological organism is that while the entire entity never shuts down and keeps going, the heart keeps beating from birth to death. In that sense, the first semiconductor fab operates non-stop, 24/7, continuously from birth to death. The lifetime of the fab could be ten years, 20 or 30 years. In those ten years, the individual components of the fabs have all changed. The machines are upgraded, the walls are repainted, and the building is redone, but the fab never stops producing.
A fab shutdown is a globally significant event. One of the examples I would like to give is Samsung. One of the Samsung NAND factories was in the news because the fab shut down for 30 minutes because of some catastrophic problems. That impacted 3% of the global mass production in the world. A 30-minute shutdown has that kind of- and these NANDs go into memory, your smartphones, your Cloud, and everywhere, so a fab shutting down makes global headlines. That's how rare these events are. These fabs never shut down. They have to have zero downtime; they are always producing. Now, of course, the capacity goes up and down. At some point, it's producing way more; sometimes, parts of the fab are being shut down and producing less. This is the very demanding environment we are going into where zero downtime is real; this never shuts down. It has to keep going. In such an environment, how do you introduce novel and breakthrough technologies? That's why I like the theme of this podcast; the way we titled it is augmentation without disruption. Because indeed, and the answer is quite simple if you look at it that way, we train these intelligent agents on hundreds and millions of hours of simulated fab time.
The agent learns to do the right thing to respond to keep the factory production on track. These agents we deploy next to the human operators that are currently running the fabs and who are sitting in this kind of cockpit- again, you know the air terminal, the airline control pilot, the control tower analogy, that they've got these masses of screens which are displaying all kinds of extremely complex information about the innards of the fab that is working. These fab operators with decades of experience are sitting there and ensuring nothing goes offline. If anything goes wrong, they jump on it. Our agent, we introduce to be sitting next to this fab operator, and so to speak, tapping the fab operator on the shoulder, saying, "Hey, I can see that you're doing this. But if you try this other thing, maybe your fab will work better." We do not touch the existing flow that the customer has. That remains intact. We add a parallel flow, where we are constantly retraining these neural networks and pushing out these intelligent agents. The intelligent agents are augmenting the human operators. They give suggestions to the human operators that this is what you can do. That is how you ease these intelligent agents into the manufacturing environment with zero disruption. Does that make sense?
[00:23:23]
Ken: It does. It leads to my next question. How do you know when an organization, a client, is ready to adopt your solution? What have you seen as some of the best practices for them to realize the full potential value of minds.ai in implementation?
[00:23:40]
Sumit: Ken, it's largely a hearts and minds problem. When we started this journey, I was more focused on some basic level of digitization that the fab has to have, which is, of course, a must-have. By basic level of digitization, I mean it has to have sensors that are hooked into an MES. An average, state-of-the-art MES is a good enough manufacturing execution system. The material handling stuff has to be automated, etc. That's the basic level of digitalization we need, and then we can bolt in our intelligent agents and what they can do on top of the MES. That's as far as the technological readiness goes. I used to focus on that, but this journey has quickly taught me that it's about hearts and minds, as in the cultures. These fabs are interesting environments because the culture and the work environment in the fab are very much a product of the location of the fab. The fab in Germany will be run- even though maybe it's owned by the same overall global enterprise, but the fab in Germany will be very different from the fab in Taiwan. Everything is different in their language, the software tools they use, and the culture of running the fab.
As I said, the fab has a lifecycle of its own. It has a culture of its own. It has working conditions of its own, and changing the hearts and minds of- from the fab operators to the general manager of the fab- has been the challenge. But we can see that the wind is turning from a headwind into a tailwind because now the AI hype, in that sense, is helping our cause because we see- like from the C-suite, we can see the orders go down, that you have to explore and take some risks and explore how AI can improve the way we run our fabs, increase our productivity, reduce downtime, increase cycle time, which are hundreds of millions of dollars in value per year per fab. It's a traditional, conservative industry that does not like taking risks. Because the risk means something breaks, fab goes down, that's hundreds of millions in impact. Changing the hearts and minds, let them have these operators trust an AI agent, and demonstrate the value in a non-disruptive way. It's a thought leadership, and it's a changing of lard. We are revolutionizing the way semiconductor manufacturing is done today. The technology is ready, and we have demonstrated this value in high-volume production environments. Now, it's a matter of changing the cultures so that these fab operators can take the risk of trusting the latest and greatest in language models in predictive.
We are applying the gamut of reinforcement learning for this industry from supervised learning, which is to predict, for example, processing time or remaining useful time off a $100 million or $200 million piece of equipment. As mentioned, we use reinforcement learning to schedule and move wafers through a fab. We are applying generative AI to predict the behavior of the said $200 million tool two days, two weeks out into the future so that we can do preventative maintenance, etc. We are also looking into using language models to help fab operators interpret and understand the state of the fab. Use the language model, which is fine-tuned to be an expert in all things semiconductor fabrication plant, which is available at the snap of a finger to the fab operators if they want to query state-of-the-art or general knowledge about what to do, how to respond to various crisis scenarios, etc., supervised learning, reinforcement learning, and generative models both in terms of generating language as well as generating other sorts of time series data, which would be predictive of the output of a tool, for example. We are bringing the whole suite of deep learning techniques to apply to increase productivity, reduce resources, reduce waste, and improve cycle time in these factories.
[00:28:34]
Ken: Excellent, you've done a great job of summarizing all the reasons that we invested behind you and certainly behind the semiconductor industry in the US under these re-shoring waves that are going on. You know, we always like to ask at the end: where do you find your personal inspiration?
[00:28:55]
Sumit: You said the magic word 're-shoring,' which also ties in with my motivation for working in this field. In a sentence, the last 20, 30 years in Silicon Valley, we have watched- or maybe even longer, maybe 50 years- we have watched these semiconductor manufacturing fabs start in Silicon Valley. Right here, this is why this is called the Silicon Valley, because the world's first fabs were here. Then, the last 50 years have been offshoring, moving these fabs to the Far East to the point where now a large part of global production is in Taiwan, China, and Korea and in the Far East and now. This process of re-shoring has been triggered by geopolitical concerns triggered by other concerns. This is also the wave we are riding because this re-shoring requires a lot of automation. There is a huge shortage of skilled workers here in the US, so that's a primary growth driver, and it's also my motivation. At the end of the day, this ensures that the semiconductor supply chain's semiconductor security is very important to global security. It is driving a whole bunch of other revolutions, underpinning a whole bunch of other revolutions. I feel that with the work we're doing now, bringing deep learning to this part of semiconductor manufacturing is meaningful and impactful. It's the iceberg- we see the tip of the iceberg, which is these language models and chatbots and stuff like this that we see in the mainstream media. But there is a huge mass of this iceberg, 90% of which is below the water. That's where we are working in the background. Because any efficiencies that accrue from the manufacturing of semiconductors trickle out to every aspect of the technology revolution- that's what motivates me; it gets me going each day these days.
[00:31:16]
Ken: For those in the audience, if you want to understand more about the importance of semiconductors, I read a book called "Chip War" by Chris Miller earlier this year. It's a worthwhile read if you want to understand the geopolitics of IP around chips and the future of supply chains. Another good reason is that we invested in minds.ai. Sumit, thank you for sharing this time and insights with us today.
[00:31:42]
Sumit: Thanks, Ken. It's been a real pleasure.
[00:31:44]
Ken: It has; it's been a great conversation. I appreciate you taking the time. This has been Sumit Sanyal, co-founder and CEO of minds.ai. Thank you for listening, and please join us for the next episode of our Digital Thread podcast series. We wish you a momentous day.
[The End]
Connect with Sumit Sanyal
Sumit is inspired by:
Sumit is captivated by the limitless potential of artificial intelligence, recognizing its power to transform industries and spark innovative thought. His passion lies in harnessing AI’s prowess to tackle complex problems and boost operational efficiency, especially in the semiconductor manufacturing sector. He is energized by the opportunity to develop more efficient, environmentally friendly, and advanced solutions that serve both businesses and the broader community. Sumit’s dedication to advancing the boundaries of AI and spearheading new technological frontiers characterizes his visionary leadership at minds.ai, highlighting his relentless pursuit of excellence.
About Minds.ai:
The products from minds.ai apply supervised learning, reinforcement learning, and generative AI to key pain points in the semiconductor industry. They’re proven to deliver results that existing tools alone could never achieve.