Conversation with Scott Everett
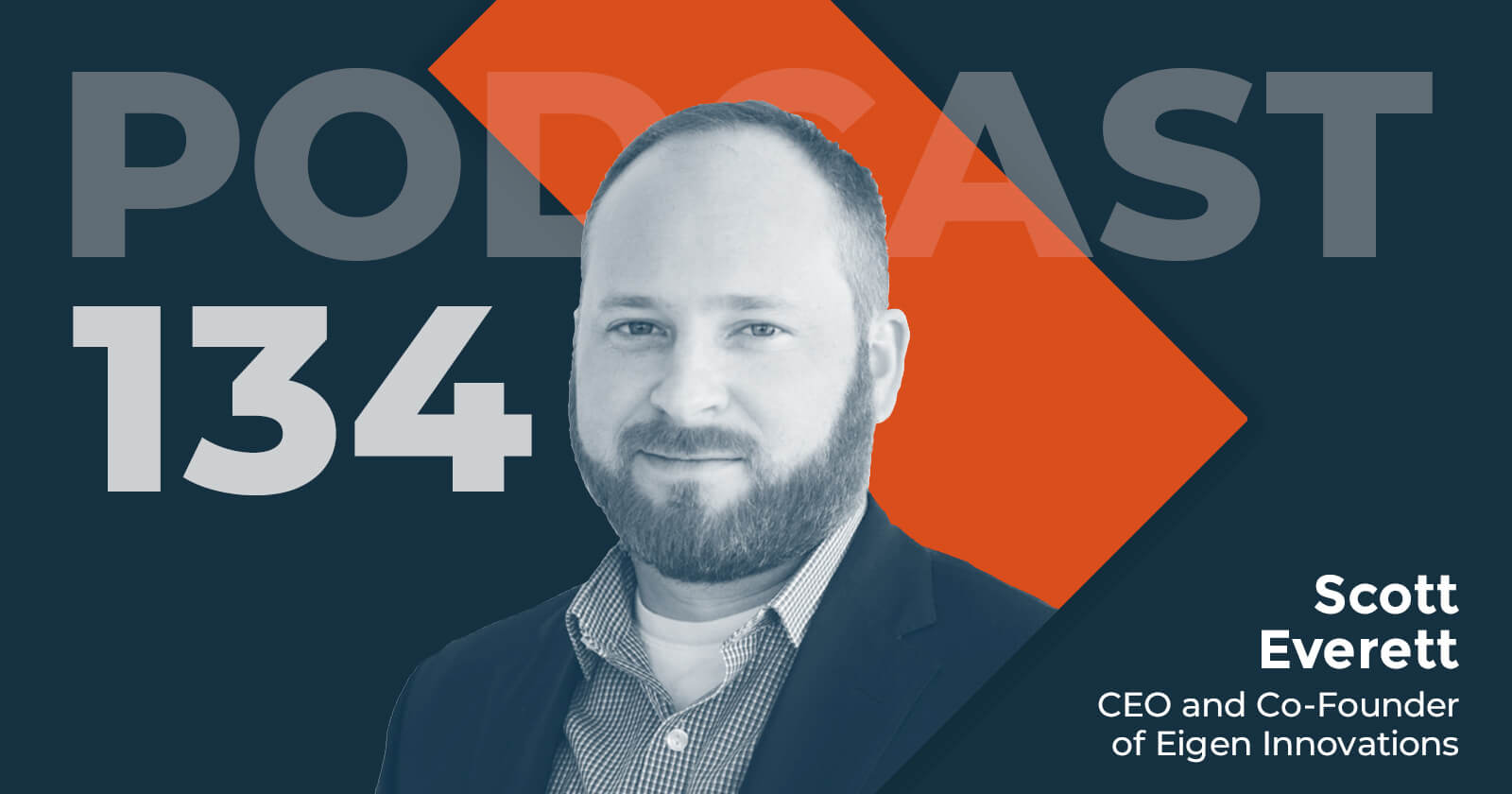
Good day, and welcome to edition 134 of our Digital Industry Leadership Series. Today I’m pleased to host Scott Everett, co-founder, and CEO of Eigen Innovations, providing advanced vision solutions for manufacturing. Eigen is also one of Momenta’s early venture investments. Scott has dedicated his entire career to solving complex engineering and quality control problems in manufacturing. He co-founded Eigen Innovations in 2012 to bring state-of-the-art technology to the factory floor, specializing in advanced industrial vision platform and machine learning. Based in Fredericton, New Brunswick, Canada, Scott spends his time working with the product development team to evolve IGINs AI-enabled solutions, as well as pitching the solution to Tier 1 manufacturers around the globe. He’s also in the process of completing his Ph.D. studies in mechanical engineering.
Scott, welcome to our Digital Industry Leadership podcast.
1:31
Thanks, so much Ken, it’s a pleasure to be here.
1:35
And it is a pleasure to have you. I know I say this because I listen to myself on these podcasts and it’s always like, ‘We should have done this a long time ago but in your case given that I think you’re like our second or third investment, I’m not sure what took so long, I know we did a blog a while ago, but to finally get down and have a really good conversation around manufacturing is well overdue.
So, I always like to start a little bit of understanding your own digital industry journey, if you will. What would you consider to be the red thread through your leadership journey?
2:09
It’s kind of fortuitous I think in a way, and I know a lot of startups the first idea that they begin with, they pivot a bunch of times until they figure stuff out, but when I look at where we started the idea for Eigen started at university with myself and my supervising professor, Dr. Ricky Dubay. So, we were researching advanced controls, and working with a number of industrial companies largely in the plastic space, injection molding, and at that time I was starting to learn about machine learning and the accessibility of data from all of these processes was becoming more and more digital, obviously. So, we were just observing on a frequent basis the fact that you would walk into these factories that have a lot of advanced automation, but they were still controlling, and tweaking, and tuning based upon intuition, and the amount of data that was readily available and accessible to help inform that decision just wasn’t there.
So, it’s interesting because this was pre-IoT, pre-AI, and we said there just had to be a better way. And so, without fail it seems every time we engage with customers that’s still a pretty real challenge. So, it just keeps me coming back to the fact that manufacturing problems are very complex, and to be able to use information in data takes a really robust solution to put something in the hands of the factory floor that’s really going to be useful and beneficial to them. So, I think that’s what I’m most passionate about, is there’s a lot of technology that’s hyped, but how do we get it into a practical state, and really have it impact and disrupt what manufacturing I think can become.
4:18
You’ve been at this for almost a decade since the founding of Eigen Innovations. I only say that because Momenta was founded in 2012, so we’re starting to talk about the decade, right?
4:30
Right.
4:30
I think you said it well a moment ago, in some sense you’re blessed in the sense that you have spent pretty much your whole professional career on this very complex and difficult problem, and thus the founding of Eigen in 2012. When you guys founded it, what problem were you trying to solve, and really for whom?
4:56
When it boils right down to it, the real problem that we saw was the gap between quality control and process engineering. And so over the years, we’ve built an industrial vision platform that’s specifically and uniquely built to close that gap. And even today process engineering and quality control are two very separate, or at least autonomous entities within an organization focused on different parts of the problem. Quality control is really often focused on mitigating and managing the risk of quality after the product leaves the factory and preventing anything that happens within the factory from getting out.
And so, we’re really trying to foot that equation where you’re using quality control information to actually control and optimize the process, rather than just applying quality control to a process after the fact. In auto motor manufacturing what we see a lot of is quality tests and the type of testing that’s required is usually at the end of the line, it's off-line, typically you’re sampling a few parts around the day, and in some cases, you have to actually destruct all of that product. So, it’s very costly, it's very low-resolution from a sampling perspective, and so the information to feedback in real-time upstream is just not accessible.
So, that’s where we’ve been using vision systems and really all of the data of the process itself, basically to contextualize the problem so that you can start to create the predictability about what’s going to happen, based upon what you’re facing in the factory in any given moment.
6:57
To describe the concept a little bit more, tell us about some of your key use cases and wins.
7:05
Yeah, that’s been part of the fun of the journey I guess for us, our wins are related to our customer wins, and I think obviously everybody in the field of machine learning in the IoT space is very aware and familiar with pilot purgatory. So, as you evolve and get to a place where you’re actually breaking out of that and creating scenarios where the technology is saving real money and having a real impact on the business; for us, those things have often really kind of taken shape in changing the whole quality assurance process. So, I mentioned destructive testing, we do a lot of work on safety-critical components, plastic welding is a good example of the process, and like I said that information about quality there’s a very strict control plan that’s determined between the tier one and the OEMs, their customers, it’s all based upon destructive testing and sampling. And so, what we’ve actually been able to start to do is completely disrupt the nature of how quality control is done and eliminate a lot of the costly expense. So, what we end up with is a scenario and solution that’s actually measuring and predicting the quality of every single part, so you’re not just sampling parts of products.
And when you create that traceability across to every single part, not just a few that you sampled at the run of the day, not only are you reducing the cost of the destructive testing, but you’re creating this really rich set of data that just helps you resolve any issues quickly, it helps you understand changes as they happen, keeps people informed. So, when we really broke through and started to see those solutions that we're bringing that real value and then starting to scale through the organization, that was in the last even couple of years, that’s been a really exciting time. And I think that’s what the industry needs more, really disruptive used cases, not just incremental improvements on what they’re already doing.
9:27
As I recall, some of the use cases over the last couple of years, there’s been both some on the process side, process manufacturing, and discrete. The process one I thought was interesting, because I remember you guys were detecting early defects in large paper rolls as they were being manufactured, and on the discrete, things like checking the quality of defrosting in an automobile coming off the assembly line, right, things like that where both the vision and especially infrared vision came in to really create a whole new – let’s say the view of the process.
I was joking earlier about our near-decade experience here, but I recall you standing on a stage at what was the first IoT World Conference, remember, this little, tiny low key we had it in Palo Alto, and I think it was a couple of hundred people in 2014. But I remember you promoting manufacturing analytics using vision, and while so much has happened, you mentioned AI and IoT, since then it seems that we’re still pretty nascent in using vision to analyze and improve manufacturing. Why do you think this is?
10:42
Obviously, a surprise to me in a way that it’s taking longer, there’s a lot of factors. What we learned from boots on the ground experience when we first really started developing products, is that a lot of the infrastructure just didn’t exist for a true vision platform at the time. So, we spent a lot of time actually creating the capabilities of capturing really unique data sources, using vision systems. You talk about thermal imaging, that’s a sensor that we’ve got – I would say probably the world-class experience in using infrared technology for process control, and it took time to really build everything around the solution, the integration to the OT platforms.
But more importantly, I think one of the big things with vision system data that people might not realize, it’s the fact that we’re really getting into a world now with vision systems where we’re trying to make subjective decisions, talk about closing this gap between process and quality. It’s one thing to just detect a very known defect in manufacturing, but it’s another thing to interpret a quality variation that’s kind of on that boundary, and the context of how human operators make those types of decisions, it’s very nuanced. And so, what we found that became really essential to take vision systems to that next level is, a) you’ve got to collect the right data, so we use a blend of sensors, cameras, but then also the sensor technology, and then you’ve got to create the flexibility to be able to respond to what the factory needs at a given point in time.
We get a lot of questions about how many training examples does it take, and how long does it take to… the world of OT is still very much used to automation technology, where it’s a ‘said it, and forget it,’ and I think when we see the truly disruptive use cases it’s how we go from just that static system to something that’s actually interpreting and adapting, and that’s a whole different paradigm shift. So, I think it's technology-driven, but it’s also the cultural shift of having the team understand what it's actually needing to get out of the system. And so that’s been part of that whole process of combining those things, to create an integrated solution where the data is highly relevant but making decisions that have a high value.
13:43
What I thought was also very interesting about your solution at the time, is what I think you used to call your Pinterest display. But think about it this way, providing a whole bunch of snapshots of the current process to an operator, who effectively is training the system by acknowledging which ones are acceptable, versus a potentially challenging condition, so the process. And the way that you did that training and then used that to augment the automation systems that were there, I thought was brilliant. And we’ve used it as a pattern for looking at other similar companies that you have kind of the same thing, like operator-led, cloud training that effectively runs on the edge, right near the camera in your case, or the sensor. And I think that model is particularly brilliant, but I love the way you guys displayed it and I think you still coined the term ‘Pinterest display’!
14:36
Yeah, and here’s what’s interesting, I mean you talk about the cultural adoption factors that play with OT, so we built a cloud platform and had some of those experiences, but it’s really hard to get people to adopt a completely new pattern in their work world. So, we actually had to take that experience and put it in line with what their typical pattern would be in the factory. So, we actually created HMI experiences that are right on the machine for that same paradigm, where it’s like we’re showing a highly visual representation of what’s going on, and we’re immediately trying to capture their feedback.
So, if you want to be very effective by thinking I adapting the system, you’ve got to capture people’s attention, there’s an obviously attractive easy side, that’s fine, that’s sort of my motto based upon a book by James Clear, but make what you want them to engage with obvious, they’ve got to have a satisfying experience in terms of giving their feedback. And if that whole thing is right there in their world, they make the decision quickly, that’s really what we found is important to get good quality feedback, which we know is so important for good quality machine-learning and AI.
16:07
Yeah, this conversation actually kind of triggers another thought relative to IT-OT. I was on a conference panel not that long ago where I had an analyst next to us kind of bemoaning that the industrial IT had not met the expectations, because they hadn’t hit 50 billion devices, right. Having come from OT I was already cringing at the point, but I think a lot of analysts think that for some reason the industrial IoT has not lived up to expectations in terms of adoption. Where do you think that mismatch might be?
16:54
We’re fortunate in I would say the place that we’re at now, there’s always that question mark of how you force adoption faster, and honestly, we’re kind of not too fussed about the IT-OT divide. What we found is most important, really the gap like I mentioned earlier, is between the process engineer, process engineering teams versus the quality teams. Process engineering is very focused on reacting to whatever the main fire of the day is. Quality engineering, they’re the ones that are looking at the stats and the data of quality, and how it's changing over time. And when you can get those two teams working together, we just saw this huge change and shift in the adoption rate and the cultural perception of what we were trying to accomplish when quality became engaged. And then once all of a sudden, you’ve got process engineering and quality assurance, they’re all teamed up, it becomes the new way that they actually manage quality and manage their process. Then it forces the need for all of the connectivity because it’s just such an efficient way to manage and solve those problems. So, I guess yeah, our view on it is the gap is largely in connecting those people.
Look, I have a lot of perspectives on data analytics, you mentioned my vision in 2014, I think we’ve been really focused on IoT at digitization of the data. But we’re not really as focused on digitization of knowledge, and there’s a big difference because if you want to motivate action, and you want to drive adoption, and you want people to be making data-driven decisions, you’ve got to tell a story. That’s why I go back to that contextualization and why that’s so important, you’ve got to create the type of consolidation of the information and have it be very contextualized to the fire, the problem that they’re facing, and presented in a way – I like to think of it more as creating a data narrative, not just data analytics. If you tell the story of this is what’s happening and why, and here’s what’s going to happen if this keeps on, and make it a very simple story to understand, people will really start to use that information in different ways. So, I think we’re just at the beginning stages of thinking about digitized knowledge versus just digitized information.
19:45
It was ThingWorx who coined a term way back when; people, systems, and things, in describing what the IoT truly was, or the industrial IoT. I know we often talk about the people element but it’s those systems that sometimes I think we don’t pay as much attention to, and those are certainly not just the automation systems but just the workflow – as you say kind of cultures if you will, and work rules. And also, it’s kind of interesting to see that that still represents kind of the challenge to bring all those together.
Speaking of challenges, at the time we’re recording this it looks really good that we are finally hopefully, powering through the third wave of COVID here, and certainly helped by the vaccines and probably just a move to warmer climate again, but what impact have you seen on this? Are you operating, and I guess going forward – we’ve called it a great digital accelerator, are you seeing the same thing on your side?
20:54
Oh, without a doubt yeah. The automotive sector which is where we’ve been primarily focused on the last little while was hit pretty immediately, and we just saw plants turned down just in the unknowns of everything that was happening. But at the same time, there was I think opportunities for people to take a step back and think about, all right how do we reorient ourselves? We’ve had some very-very old factories that you would think wouldn’t really be at the forefront of the digital revolution, but they said look we’ve got to basically take this 100-year-old factory and be able to operate it remotely. So, it definitely changed the attention and the urgency I think that was being placed within the organization on the types of use cases and applications that we’re developing. We’re seeing a lot more… the actual infrastructure of these organizations now has the type of titles and roles that are specifically focused on what the new wave of manufacturing is going to look like. So that’s all just compiled to a real acceleration, and not even what would probably take months in terms of just getting the stakeholder/organizers in an organization seems to be happening much faster.
22:29
I think it goes back to what you astutely said earlier, ‘Digitization of knowledge’ and especially we talk about the challenge with knowledge workers leaving, and certainly COVID has probably accelerated even that a little bit, and how do organizations retain that knowledge. I know of several companies really trying to focus on that, but clearly industrial IoT in many different ways as you said, it’s not just about the data itself or the sensors, but the knowledge that goes with how to utilize those to affect a greater process. So, because you’ve talked a lot about industrial vision, I’m going to put you on the spot a little bit now and ask you to put on your industrial vision goggles. What do you see for the next five years in digital manufacturing?
23:24
I really do think the challenge with the way IoT is rolled out, we’ve created a lot of smart systems. There’s an article that I was reading a little while ago that was talking about smart systems are capturing data and just sort of reporting the news back, but it created all of these different silos. And if we want a truly intelligent system, in our field we’re really interested in the progression of what we would call transfer learning; so how do you take that digitized knowledge that some machine learning and a team has accomplished on one machine, and then be able to replicate that within a fraction of the time and the effort for the next machine, and the next machine, so that your investment in an application within an organization has a compounding and scale effect.
And so, pulling down the walls, creating more integration in the processes between the process quality divide, it's quality-controlled not a quality control anymore. So, I think that’s just going to lead to a lot of really interesting options around the flexibility of manufacturing. The automotive industry has always been interesting because they’ve got these refresh cycles on all of their vehicles, so they’re constantly retooling and redesigning. I’m really excited about the fact that these high-resolution streams of data such as vision systems and video analytics, like some of our applications are actually short video clips of every single part that we’re pulling out information from, tearing down the walls across the stack to be able to create a lot better contextualization of all of this information, because I think that’s really what’s going to allow for that scalability.
What you learn on one application can only be applied to the other if you’re able to accurately evaluate and measure all of the differences between the context from one machine to the next, even if they’re very very similar. And ultimately in the long run Ken, I’m really excited about what I think is going to become more distributed manufacturing. With digitizing knowledge you can apply that wherever, and so it creates a whole new potential in terms of how factories are oriented, and how we even think about designing factories; are we going to be capable of manufacturing in more local settings to the market, rather than having to ship product all over the world? Those types of things.
26:12
Yes, especially going back to one of the impacts of COVID that we’ve seen, onshoring of manufacturing has been, certainly in the US, a big topic as of late. We just featured on our last podcast Hans Hanspal who was really kind of focused on that topic as well.
So, IGAN was Momenta Ventures' first investment in a Canadian company, and as a Canadian entrepreneur, I suspect you’re a believer of what a great place it is to do business. What makes Canada such a great place for startups?
26:51
We have really cold winters and I think that builds in a natural resilience!
26:58
I’m sorry to interrupt you, but I remember at one point in my life I interviewed at Microsoft up in Redmond Washington and they told me the same thing, ‘It rains here all the time, so it’s a better place to code’!
27:10
Yeah, that’s one thing I’ve learned, you’ve got to have grit, you’ve really got to dig in and hold on. So, honestly, it’s been really interesting, I’ve just always appreciated the fact that there’s a lot of really brilliant individuals coming from rural Canada. I’m from a farming family actually, eight generations, and I just love the opportunity to be able to take my love of machines – I was obsessed with tractors when I was a kid – and to see the connection from those very basic things that I was always so passionate about, and to be able to create a company that’s very high-tech. There’s been just a really great lineage, especially in the field of machine learning and artificial intelligence, throughout Canada and the research institutions. And so, there’s a lot of resources and networks here that have been really foundational, and just helping us along.
Honestly, in the early days it was just having people who had been in the field of artificial intelligence long enough to believe in us, and just say, ‘No you’re actually not crazy like everybody else probably thinks that you are, but there’s a huge opportunity here.’ So, yeah that culture and that support have really helped us along our way.
28:46
Yes, and we’ve invested in a number of other Canadian companies, and we’ve heard pretty much the same thing, it’s just a great ecosystem to support startups in there.
I guess given your success and beyond recommending somebody move to a ‘Cold climate’ what advice would you offer to aspiring entrepreneurs?
29:13
I think for me the cliché things that everybody talks about are very true. I believe that No.1 you’ve got to surround yourself with good people, so finding mentors who are there for you, and people who are actually investing time into you and in your journey is really important. I think one of the most important things that my mentor and network helped me in the early days was to figure out how to build a really great team.
So, culture, I can’t talk enough I guess about the value of team culture and being deliberate about that culture, and then what happens when you start to see a team come together. I think that’s one of the things that is interesting about start-ups in the IoT spaces, we’ve had the agility to build what I think is probably a good template for the factory of the future. We’ve got this blend of IT-OT, process quality, machine learning, all in these highly effective teams, and it’s pretty incredible to see the types of solutions that they can tackle and what they can take on.
I think the third thing, you really can’t get around the fact that you need to wrestle with the fundamentals of your business. You can hire people to come in and give you a perspective, you can lean on people, but you still need to wrestle with it for yourself, and so there’s an element of not getting caught up too much in the hype of the startup world and protecting your time and attention so that you’re focusing on those deep problems, be it technical, culture, customer, market-related. I think you can waste a lot of time, and I think it is really important as an entrepreneur to recognize that the deep work and the focus thought that you can put in, is as important as all the busy activity that’s pretty easy to get wrapped up in sometimes.
31:24
That’s what I’ve always loved about being in startups, or being in young companies is, as they say, you’re close to the metal! Fair metal in some sense. And there just isn’t a lot of time to waste a lot of cycles, otherwise, you’ll find yourself back at a corporate or doing something else. So, it keeps you real, and literally, if you’re not waking up every day thinking, ‘Oh gosh, how am I going to make payroll this week?’ then you’re probably not as close to the metal as you may need to be. So, it’s as you said, I think earlier you used the word grit, if I remember right, it requires a healthy dose of that with a capital G.
Finally, a question I always like to ask, generally what inspires you? Think of speakers, books, video series, whatever the case may be.
32:23
I’ve been really fascinated lately with truly prolific creative people, one of my favorite shows I guess has been Chefs Table on Netflix lately, just because you get to hear these stories of people, and I think it’s the grit factor that’s inspiring. But I’ve come to just appreciate the fact that truly creative individuals are very disciplined, like going back to that you’ve just got to put in the cycles. They consciously shape their lives, their worlds, and their environment so that they can maintain a high level of focus and discipline on just honing their craft. When I think about engineering, I really don’t see much separation in that. It can be such a rewarding and creative process to come up with the solution for a complex problem, and really that’s just a function of staying focused and putting in those reps. So, it just always impresses me I guess to see people from all these different domains, and that common thread seems to really be the underpinning of their success.
33:35
And clearly, yours as well for almost a decade!
33:40
Yeah, well hopefully. I think this journey, it got a lot of legs, obviously, we’ve been through so many different cycles, but the technology and everything that’s changing so fast is just opening up these, I think, whole new ways to think about the way that we’ve done manufacturing in particular. So, it’s a journey I want to stay on for a while.
34:05
Well yeah, and if anything, I think you guys are hitting truly a good velocity in that regard. I remember back when we were first talking, the things you had to do full-stack and learn and build, how much of that now is available more or less off the shelf. So, you can really focus on the true value-add in terms of your overall solutions and algorithms and such, but it’s probably been the story of most of the industrial IoT if you will, companies that we’ve invested in. In the early days you had to do everything, everything full-stack, right?
34:42
Absolutely.
34:44
And so, if anything now, having those deep relationships, knowledge in the industry, knowledge of the people, systems, and things, all of that now allows you hope to move much quicker on COTS, Common Off the Shelf – systems and components. So, it’s interesting.
Well Scott, thank you for this long overdue and insightful interview today.
35:08
I really appreciate it. It was fun to chat, it’s great to catch-up. I remember that first conference Ken, they pinged me on LinkedIn and I thought I was being catfished, and so I went back to it four times, ‘Is this really a real thing?’ And so, I was like, ‘Okay, I’m going to take a chance,’ I jumped on a plane and I ended up in California, and that’s where we met.
35:30
Yeah. It’s how I guess relationships are built over time. It seems like it will be happenstantial at times, but I always kind of believe things are meant to be and guided in that regard. But I must say, that was one of the funniest conferences in the early days of industrial IoT.
Well, this has been Scott Everett, co-founder, and CEO of Eigen Innovations, and bring the vision to the industry, if I can tagline it that way. Thank you for listening and please join us next week for the next episode of our Digital Industry Leadership Series. Take care and have a great day.
[END]