Conversation with Simon Kampa
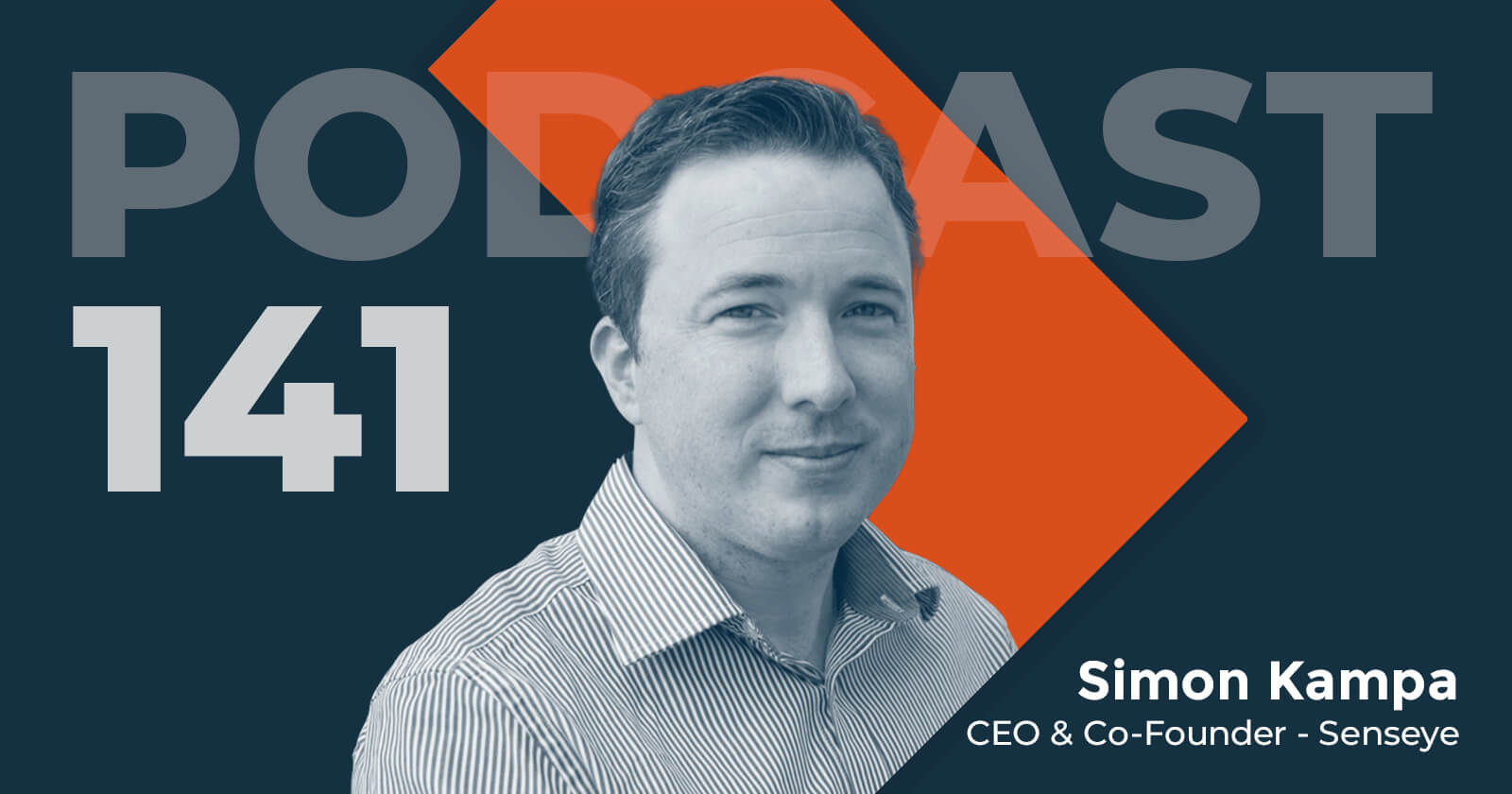
Good day, and welcome to edition 141 of our digital thread podcast series. Today I’m pleased to host Simon Kampa, the CEO and co-founder of Senseye, the leading cloud-based solution for predictive maintenance 4.0. Senseye is one of our Momenta portfolio companies.
Simon has over 20 years leading the development of mission-critical software, across industry, aerospace and defence. Before Senseye he was managing director of critical software UK, where he expanded its operations and established its focuses on data analytic systems for complex machinery, such as helicopters, aircraft, and trains. Simon then co-founded Senseye to disrupt the predictive maintenance domain with a fully automated and turnkey solution targeted at the wider industrial sector. Simon holds a PhD in Computer Science from the University of Southampton.
Simon, welcome to our digital thread podcast.
1:27
Thank you, Ken, thanks for inviting me onto this series, always a pleasure talking with you and the team at Momenta.
1:32
Yes, as well. I know I say this all the time because I listen to my podcast, and I always say it, it’s finally time that we had you on there. But you were one of our earliest investments, so it absolutely applies, I don’t know why we’ve waited so long, but I’m sure we’ll have much better stories for that as well. So just in starting, what would you consider to be your digital thread, in other words, the one or more thematic threads of experience that have defined your digital industry leadership journey?
2:03
I guess from when I did my PhD it’s always been about data and extracting value out of it. If I look back at my first career step which was at Lockheed Martin in the defence industry, it was very much about analyzing interoperability and data between different military assets; how do tanks and fighter jets exchange situational awareness and other tactical data. The next position I took then is even more about data, it’s about analyzing London vehicle data, so this is back in… it must have been the early-2000’s when the congestion charge came out in London, and you had ways of analyzing all the vehicle data, and start charging people and collecting evidence, it’s all about again collecting data.
Then I went onto a Home Office project which was about analyzing data on what’s called a lawful interception, or voice and data calls, aka wire-tapping – so I can’t talk much about that one! That was about looking at huge quantities of data and extracting value from it.
Then my final position before later, setting up Senseye, which really set the foundation for Senseye was working at a company called Critical Software, and predicting failures in complex aircraft and helicopter machinery, such as imagine a helicopter gearbox, a very complex piece of machinery that’s put under incredible strain, and it’s emitting a huge amount of data. Again, it’s about extracting value from that data and giving insights into what’s happened to that machine.
So, I guess my digital thread going all the way back to my PhD, and then my career up until today is all about converting data to insight.
3:57
And that is a really good lead into our conversation today as well. So, since you mentioned the PhD let's go back to the start there. I noted that was in the semantic web which certainly was a hot topic and remains one. What was the semantic web and what were some of the insights from your studies around it at the time?
4:17
Well, I’m really pleased that you’re familiar with the semantic web. I think it was at the turn of the century a massive topic, and it still has its followers and influencers, but perhaps the term itself is not so well-known anymore. It was coined back then by Sir Tim Burners-Lee who invented the web, and he realised that the web was essentially dumb to machines. Machines, computers couldn’t understand what the content of the website was about, and the web traffic was about, and so it was difficult to offer advanced services on it. The vision by Sir Tim was always to recreate a smart web where you can have very smart search engines, and automated servers and things. So, my PhD was in that broader field, and applying basic artificial intelligence-type technologies to demonstrate the possible; we had smart web, what could we achieve?
But there were I think two outcomes that happened from the Semantic Web. I hesitate to say that ultimately, the Semantic Web failed, as I don’t think it did, and it did really contribute to advancing the art of possible and has morphed into other initiatives. Nevertheless, two of the big outcomes I think, first Page and Brin came along and founded Google in the 90s. I remember coming across their stand at my first academic conference in ’98 in Amsterdam, and they were giving away free merchandise and whatnot. At that point, I switched over to Google from AltaVista if you remember that old search engine and haven’t stopped since. But I think what Google proved and this kind of disproved the Semantic Web, that actually brute force and lots of processing was a far more pragmatic solution that derived surprisingly good results. Sematic web required quite a barrier to entry by adding a lot of metadata and other intelligence to the web, and Google I think proved actually we can be pretty good without doing that.
In a way as an aside, and maybe we can mention this later as well, I would draw some parallels with that sort of pragmatic approach with our philosophy at Senseye as well.
I think the second reason why Semantic Web maybe didn’t get the uptake that some would have liked it too, it does add a significant layer of complexity. The beauty of the web is it’s so simple, and it’s so accessible by everybody, and the Semantic Web went against that ethos and added that layer of complexity, added that barrier to entry. And actually, people were happy with good enough and the Google approach, and things like that. So, it’s a great thing to learn from Semantic Web and it still influences thinking today, but I guess it doesn’t really exist in the way that Sir Tim Berners-Lee wanted it to.
7:20
Certainly not in the sense I guess that there are explicit things – I’ll use the term, tag, to simplify the idea of Semantics, versus the idea that things are discovered and self-identified in tag, think of XML in some sense. And I agree with you, I think the explicit approach didn’t happen, but that implicit one is where the power is in many things that we do, and especially when you think of the Internet of Things, and this idea that devices are discoverable and their capabilities are discovered, and then their insights are discovered, as all part of the interrogation if you will, with the devices. So, I agree with you, I think it’s a great lead in to the work you guys are doing.
I know you went on to lead enterprise great development efforts, Lockheed that’s also my alma mater, Siemens and then finally ended up as Managing Director of Critical Software UK, a term that may be the audience has forgotten here too, a CMI Level 5 company. As I remember it was only IBM at the time for the Space Shuttle computers that had reached that pinnacle if you will. But I know you guys are providing safety-critical software engineering for Aerospace Energy Defense, Government, and transports. Tell us a bit about some of your early wins at Critical Software, and I guess really what inspired your interest in predictive maintenance there?
8:43
Interestingly, you mentioned CMI Level 5, I think it’s still living with us. When you’ve worked at a company that has that for six years, you are a very deterministic almost process-driven business, and you have to be because you’re developing safety-critical systems. And even today when we think well actually, we’re a quite dynamic start-up with process light, a lot of our investors still say that we’re quite process-driven compared to others in their portfolio. So, I guess we can’t quite shake it off.
But from a personal development perspective, it was my first role as CEO MD, and that’s good and bad; so, you obviously get to learn the ropes on someone else’s dime effectively, but then at the same time I think some of the best founders have done it as a first job unencumbered by the corporate way of doing things. I think we certainly noticed that in the first year at Senseye, we had a little bit of that corporate overhang when we first met you as well Ken, that corporate overhang that we had to unshackle ourselves from and become free in the way that startups operate.
But during my time at Critical over the last six years, I hired my three co-founders, so I guess that was the best thing I did there, and in particular, I hired Rob Russell who is our CTO, and his background has been in aerospace for the preceding 18 years when I hired him. He became the CTO of the newly established – I can’t remember actually what we called it, like Data Division I think, CTO of Data, which was essentially tasked with expanding our critical predictive maintenance operations across Aerospace, rail and other critical industries. It wasn’t called predictive maintenance at all, that’s a newer word, it was terms like diagnostics & conditional monitoring, and prognostics were used. But essentially it was predictive maintenance, and that really got us deeply into that world.
We worked on several really interesting projects for the largest companies, GE, Airbus, Boeing, and helicopter companies like Leonardo, Eurocopter, Sikorsky, as well as rail companies, and you’re working on some really critical projects and any failures on these high-value CapEx equipment’s leads to a loss of life, so you do feel that you’re working on something really special. I think that’s what really got us into this space, and we always had a vision of, this is great doing this technology for the big guys on big machinery, but can we take those concepts and that capability, and actually offer it to the wider industry who previously had been kind of locked out, based on price fundamentally, and also the complexity of having these systems. I think that’s why we then decided to set up Senseye. So, yes, critical was the inspiration to Senseye really.
11:58
I think we first met you guys probably in 2014 or ’15 as I remember at the time. You talk about the corporate overhead, as I remember I think the initial challenge simply was just finding product-market fit. You guys were looking across several different sectors, some I would say biased a little bit by grant work you were doing at the time in UKTI, but ultimately was finding that sweet spot of which you clearly did in that time.
You mentioned Rob Russell your CTO who also co-founded Senseye with you, and I believe also at the time your marketing head, Alexander Hill who also co-founded Senseye as well. So, one of the first companies that we knew of really looking at this idea of bringing condition monitoring, or as you say prognostics, or making it more accessible if you will to other enterprises outside of the traditional defence. So, when you guys left Critical Software to find this, what problem were you truly setting out to tackle, and really for whom in terms of your target group?
13:06
Well for the first six to nine months, and I think it culminated probably when you visited us in Southampton Ken. I guess we did the classic mistake of startups where you start at the vision and we were going to solve the world, every vertical we wanted to tackle, and clearly you can never start in that way. So, we wound it back to where the obvious place was, which was looking at machinery in the industrial space. We even had a spell in agriculture you might remember which was actually grant work, and that’s probably the downside of grants in giving you money to follow the wrong things. But by the end of 2015, which was our first year, we had narrowed it down to looking at predictive maintenance, it still wasn’t a word in 2015, within the industrial space and particularly within a few sub-verticals.
But the problem we were trying to solve was taking capability from the higher-end sectors like oil and gas, aerospace, railways, where very-very unique bespoke approaches, you’re developing physics-based models, hundred-million-dollar projects and very skilled engineers using those tools, and said can we create the same curve capability but, and it’s a bit of a corny word, but democratize it, make it available to the wider industry who may only have motors, pumps and things, and offer them a similar capability.
To do that you need to bring the cost down by several orders of magnitude, and that’s obviously where AI and things come in because you can start automating it, but also making it accessible to your average engineer, not a skilled diagnostic engineer which may be commonplace in Aerospace oil & gas, but most industries haven’t got those skilled engineers. So, we had to develop a way of automating something very complex and then making sure the output and the user experience is as simple as possible.
So that’s where we were at the end of 2015, and thanks to some clever people, including you guys at Momenta, we narrowed down our product-market fit. We’re still tuning it, every six months we still challenging ourselves and tuning it as you need to do, but the biggest pivot if you like was at the end of 2015 to make sure that we really started to focus.
15:42
You know, as I look at the other use cases you were looking at, I think Agtech as an example, in some sense the markets themselves are coming to you in terms of the availability of use cases, where the initial focus I think is absolutely really important to have and really proven to tune your hypothesis about your value-add. In some sense, I think the wider remit of the things you guys are looking for were original will come to pass, and it’s a matter of those markets just getting to the point of ready. I can certainly vouch for Agtech because we’re doing quite a bit of Agtech work lately, which is almost reminiscent of what you guys were looking at in the first place.
I had to laugh, you said the word democratize was a bit corny, but in some sense that is what Moore’s Law truly is, it’s the democratization law in some sense. And in IoT, you’ve seen that especially where what was here-to for SCADA Systems Plc, things like that are being done in some cases are doing low impact, if you, will use cases. So, you naturally see that democratization coming down to all things OT or IoT in that case.
Tell us about some of your notable use cases and wins at Senseye.
Well in the first days, the first year, it was trying to get access to machines and data, so that’s almost desperately you need to charge for it, so luckily, we had some local companies that were willing to do that, and that was the start, but they were never going to be our customers because our sweet spot was always going to be the very high-scale diverse factories. That in itself is a bit of a problem in emerging industries where the initial use cases are often limited to small scopes, factory of single machines and things. But in terms of our first notable win, this was in 2016, was with Nissan in the UK, and the timing just happened to be spot-on for that because Nissan at that point had strategic ambitions to increase the productivity of their plants, and the UK plant, in particular, had been given the remit to explore that.
They had a couple of failed projects with your typical sort of big industrial automation businesses, and then they found out about us. It was just perfect timing because they were under pressure to prove something, and they had two failures already and then they got this nice UK startup with experience in Aerospace and things that just clicked for them. So, there was a huge pull from them, they unusually had a lot of digital data already, this is back in 2016 and I still think within the automotive industry they’re an anomaly in terms of having so much of the factory covered by digital equipment and collecting sensor data.
So that was our first really big win, and it continues to be a great account that keeps going from strength to strength. And then soon after, maybe six months after that, so this is probably the end of 2016 we secured our first, if you like, really competitive win with a major steel producer where we were pitted against twelve other vendors, including the usual big guys, incumbents, and some of the younger competitors. I was like, ‘Wow, this is going to be a tough one,’ Alex my co-founder is more naturally optimistic, he was like, ‘Great, well we’ll do this.’ And we won it hands-down! And I think that’s a great marker for us to say, hey we’re okay, we can complete here, we generally have a product that has been produced by a much smaller team, at that time it must have been 15-20 people, and we can compete with some of the big guys. So that’s a really major milestone for us to give us that recognition and credibility that we were serious players.
19:35
You’ve used the term predictive maintenance 4.0, or PDM 4, to describe your solution space. What does the term mean to you, and how does this differ from condition monitoring as an example, or prognostics?
19:49
Alex came up with that term and I quite like it. For us, predictive maintenance 4.0 is really covering entire factories, not just limiting the scope, and if you look at Industry 4.0 it’s all about big transformation project and covering your entire supply chain and your factories, making it more accessible to your average engineers, to your leadership team. We see a lot of predictive maintenance projects that are so narrow in their focus that we wonder – there’s a lot more impact they could be having. So, Russ has always said about that massive scale and integrating it with so many other Industry 4.0 systems that don’t have them in silos.
In terms of how it relates to all those other terms. For me and us, predictive maintenance is the sum of diagnostics, prognostics, condition monitoring, and prescriptive analytics and things, no one technology suits the solution, so it’s a combination of all those.
21:03
Let’s drill down on some of those terms because the audience may not be up to speed on that, and I think you guys have developed some nice semantics there. So, as I understand it, and correct me if I’m wrong if I look at industrial analytic, I’ll call it maturity, there’s descriptive analytics that kind of tells you what happened in the past, a dashboard if you will; diagnostic analytics, helps you understand why something has happened in the past; predictive analytics which we’ve been speaking of, so predict what’s most likely to happen in the future. And then the kind of nirvana in the industry is prescriptive analytics, so recommending actions you can take to affect those likely outcomes.
Do you generally agree with these definitions, and if so, when do you see us arriving at this pinnacle of prescriptive?
21:51
Yes, I’ve seen those and similar descriptions in the media, in the press and other places. I can’t say I’m the biggest fan of them, at least not the first two, I see them very much as an IT-driven take on an OT problem space. For example, I wouldn’t classify diagnostics only about looking at the past, traditionally we use diagnostics to tell about the future, you can look at the current state of the machine and identify whether it’s in a failure mode, and then you can interpret; when you think it might fail. It may not be as accurate as predictive analytics, but it can still be used to talk about future event. And certainly, in Aerospace those terms were, and still are used.
But just to expand on what I said in the previous question, for us predictive maintenance has to be all those four working in unison, it’s not a mutually exclusive list; different machines behave differently, different scenarios, you’ve got different people operating it, so no one technology approach will give you all the answers.
And as for prescriptive analytics, I would agree with that definition. There are some solutions including ours that provide some of that functionality. Certainly, Rob Russell, our CTO’s view on it, he’s a little more anxious about it, he thinks by definition its always going to be a very customer-centric approach, because each customer has different processes and standards, each one does maintenance differently, they have maybe different protocols for when to take certain actions, so it’s always going to be very specific to a customer. That then by definition makes it harder to automate and create a solution that then scales.
So, we support prescriptive analytics to an extent, but it is driven by the customer having to add some of their expert knowledge into the system, which then for us is a big challenge because we like to have a fully automated system out of the box, turnkey, and as soon as the customer has to do something it kind of negates that a little bit. So, I’d say we’re getting there Ken, on prescriptive, but maybe another two or three years away before we can truly call it that.
24:10
Yes, I guess the best analogy I’ve seen is at least on the Agtech side since we mentioned earlier the idea that instead of just predicting a crop yield, based on environmental factors and such, you actually in some sense inform it by telling you what seed to plant, at what point to fertilize, what point to water, and of course, that goal setting, if you will, is reassessed every day. So, in some sense, you achieve the yield you set out based on putting all the factors together, versus just predicting that the yield will be a certain percentage, or perspective if you will.
It’s pretty interesting because you start to see that coming, even in process industries we’re seeing where setting these goals is efficiency is more important, or do I have a need to drive the equipment at maybe 90 per cent of capacity, which I know will shorten its life, but will get out these six orders that I have to get out. And so, I fully agree with you that it is very much customer-driven, but it’s also very much driven by almost day-to-day, if you will, customer needs. And so, there is a whole world ahead of where we sit with predictive, but you guys I think are already building the basis for that.
Let me ask, how do you know when an organisation is truly ready to adopt predictive maintenance 4.0, as you call it. And what best practice have you seen and truly realising that potential value?
25:39
Yes, this is a massive area, and I’m pretty sure every single one of our peers and competitors has the same challenge. There’s a huge demand for wanting industry for technologies and predictive maintenance, but that demand is not always met by readiness. So, in my head I see it as, let’s say in our industries at least, 90 percent of businesses want to do predictive maintenance, and by now experience of those maybe only 20 percent are going to be worth engaging. But the trouble is, there’s a similar quote in marketing, you don’t know which that 20 percent is until you’ve spent considerable effort with them. The other 80 percent can in the meantime sap a lot of your presales effort and energy before you realize it’s not going to really be a successful project. We focus only on the largest Fortune 1000 type company, so they’re all big logos and we all want them to succeed, but a lot are just not in the right place yet to succeed at scale.
So, we and others see it as very much the two areas that we look for, to judge a company’s readiness. One is probably the most important, its cultural readiness, not just for predictive maintenance but Industry 4.0 in general. We look for is their strategic support from C-levels for such initiatives. There’s an awful lot that seems to sit in the innovation R&D arm, but don’t really have any operational support, so we look for strategic support. And more specifically we look also then for are there really good maintenance practices because if they haven’t got the fundamentals in place, they’re not even doing preventative maintenance properly, then it’s going to be harder to get the workforce to adopt a more proactive, more advanced solution. So, we look for that, and there’s a number of other factors we look for.
The second one, also important but you can negate it if the first one, the cultural one is strong, is technical readiness. Most businesses I don’t think fully appreciate what data they’ve got available, or they haven’t, how to extract it and how to share it, and even if they do have an idea, they then lack the capacity to do the job themselves. So, you can bring partners in, but the whole thing slows it down.
We’ve encapsulated these two sorts of vectors in something we call Opedium Readiness Index, which our sales teams use together with a customer to evaluate their status quo, where they are, where they’re going, and whether it could lead to a successful project; basically trying to qualify them out or in as quickly as possible, because in the early days we learnt the hard way that you can spend over a year getting closer and closer to a customer, and then realise there’s never going to be anything here worthwhile in terms of a big project because the company is just not there yet in their stage of evolution.
I saw, and I’m sure you’ve read these as well Ken, there were a number of studies that came out in the last 18-months from all the big analysts, Gartner, ABI and Forrester, about somewhere between two and three, and three and four Industry 4.0 projects don’t scale. Some of that will be because the products themselves may not be fit for purpose for the vision of industry 4, but a lot of that I think is also just businesses not being quite ready yet, and not maybe getting to grips with what Industry 4.0 really is, and what the trends underlying it really are.
29:17
You mentioned the last 18-months, and just as an aside I’m curious, what patterns have you seen over the COVID timeframe, if you will, relative to both to your business, but more importantly the use cases that you guys are really driving.
29:32
Certainly, Q2 aside from last year, what we’ve seen is very much acceleration in this space. COVID has highlighted the inefficiencies in supply chains, productivity issues and things. So those have, I would say, accelerated. The industry that we’ve probably seen the most acceleration, and the most momentum and purpose is metals and mining, wherein the midst of the worst of COVID I think last May or June, we secured another metals-mining customer, and we’ve probably secured one every two months since then. For these guys, it’s very difficult for a lot of them to turn off their equipment, these might be big blast furnaces and other very expensive CapEx-rich equipment that is difficult for them to turn off. So, their only choice really is to optimize what they’ve got, so they’ve been accelerating initiatives like predictive maintenance.
30:35
Great insights there, and pretty interesting to see the metals and mining angle on that. So, given your own entrepreneurial journey, especially starting your own company here in 2014, what advice would you give other startup founders?
30:53
My wife would say don’t do it, but I’ll ignore, and I’d say quite the opposite, do it. You know this Ken because you’ve teased me about it, I don’t think it’s an issue in the US, but in Europe, there’s probably a little bit more anxiety about setting up shop on your own. In Europe, we don’t like a failure, so I think that stops some people from taking the leap. So first I’d say just do it, I think once you get over those first two or three months you’ll never look back, and you could never ever go back to the corporate world.
But beyond that, and for us, I mentor another couple of companies and it’s the same that I say to them, it’s all about focus-focus-focus, and that product-market fit. I’ve seen so many other peers I talk to, they were like us in the early days, they’re starting at the vision trying to execute the vision from day one, rather than developing a journey to get to the vision, but first, just get a bit of proof, get the proof point that your product works, and there is a customer willing to buy it. There’s a lot of impatience out there, people wanting to accelerate that and start with the vision, so I’d say the focus word again there.
Otherwise just in general… and that’s why I quite enjoyed this exercise, get your head out of the minutiae of the business, which because you’re a founder and a CO you wear a lot of hats, and you get involved in so many parts of the business, sometimes it’s really difficult to step away and reflect. Those are the important points where you need to have that strategic time and headspace to think about where you’re heading.
And the other thing, as it's mental health week, I’ll say look after your mental health because that can strike suddenly when you’re in very stressful environments, you do need to look after yourself. I’m lucky I haven’t experienced it myself, but I’ve seen others where it silently creeps up on people, it hits them and suddenly they’re out of the business for two, three, four months, which obviously is a disaster for a startup. So, everyone looks after their mental health and take activities and actions to look after themselves.
33:04
Excellent advice, especially on the latter point, people I think generally underestimate the challenge we’ve all had given sheltering in place, COVID, restrictions, and even simple things like running out of toilet paper, right! Or the runs I should say on toilet paper as they were early on. So, it makes you appreciate the little things in life, doesn’t it? Which is a good lead to the final question, where do you find your personal inspiration?
33:35
I struggle with this kind of questions Ken, I can’t name my favourite food, or my favourite song, my favourite that… I read a lot of books and I follow a lot of different people on LinkedIn and things, but what really interests me all the time is… and we’ve been in the industry world since 2014, so I guess in some ways that make us veterans. I find it so exciting, just the whole ecosystem of Industry 4.0 how it’s been developing, how it’s still changing dramatically, and different angles are coming up. I’ve seen exciting startups come and go, meeting your fellow entrepreneurs, and see what competitors do. I think it’s fascinating what sometimes competitors think of that we haven’t, you can learn a lot from that. So, for me, it’s really that mass dynamism and buzz in the industry for space, globally, that I just find enthralling.
34:38
Yeah, we certainly do as well. I’ve always liked the Silicon Valley term, copertition, as there’s really no competition there’s just the ability to collaborate and compete at the same time, which is a nice dichotomy to hold in your mind. But that is an ecosystem by definition, everything overlaps a little bit and supports each other, and derives from each other.
Well, Simon thank you for spending this time with us today.
35:06
Thanks, Ken really for inviting me onto this. And as I said to you before the talk, when I was thinking about some things I’d like to say, yesterday, it really allowed me just to sit back and reflect on a journey we’ve taken. I think that’s a really healthy exercise. So, thanks for inviting me.
35:27
Yeah, and I think the journey as we joked earlier on your predictive versus prescriptive, I think is a journey that’s just beginning, so it is a very fun place to see. Especially those of us, like yourself who have deeper DNA, who have seen this industry and Aerospace, and other sectors, and you see all of this literally democratizing, is your term, coming down. So, there’s a lot ahead of us in this regard, and you guys are very well placed. We’re proud to be investors in you.
So, this has been Simon Kampa, CEO, and co-founder of Senseye. And if I can say so, the extractor of industrial insights, we’ll call you the miner.
Thank you for listening, and please join us next week for the next episode of our digital thread podcast series. Thank you and have a great day.
[End]