Conversation with Mario Montag
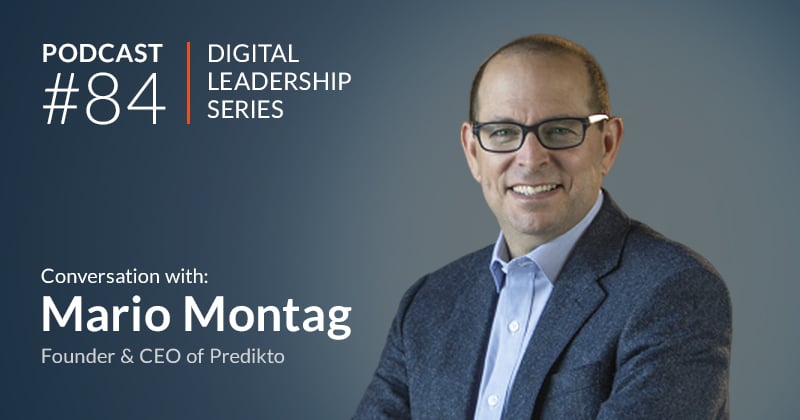
0:14 Good day, this is Ken Forster, founder and Executive Director of Momenta Partners and Momenta Ventures. I’m sure many of you, our listeners, are wondering why the change in voice here, well I’ve had the opportunity to participate in a number of live and webinar events for Momenta partners in the past. Our podcast series which were over 80 companies interviews that we’ve done, I’ve left that to others in our organization, and I have to say by popular demand I’ve been asked, chided, inferred or whatever the case may be, to start to take an active role in really driving these forward. So, I’m really happy to be kicking this one off, particularly today as it’s my distinct pleasure to introduce today Mario Montag.
1:04 Mario was the founder and CEO of Predikto, a company that we knew well, and then most recently was Chief Data Analytics Officer at UTC (United Technologies). So, what’s interesting to me about Mario particularly is, not only was he an early founder in this space of call it industrial analytics, that a space Momenta Partner’s & Ventures knows well, but also was able to sell that into a very large corporation and to get a sense of how this technology is creating both opportunity, and disruption, in that as well.
1:49 I’m going to start really quick this series and a number of others as we do this, to talk about what are called the Momenta connection, because Momenta’s very rich ecosystem between our advisory practice, which is focused on a lot of M&A work and strategy, our talent practice which is placing key executive leaders, and of course our Ventures practice which is investing in disruptive start-ups in the space, we have a chance to touch a lot of companies.
2:18 Mario particularly is an interesting one because he pretty much checks all the boxes, we took a very early look at investing in Predikto several years ago; I’m both disappointed and proud to say he was one of the ones that got away in that regard. Later on, we on behalf of a leading wind-turbine manufacturer were helping to place their analytics team. We took a deep dive on Predikto yet again, and this came up one of the top five finalists in terms of potential acquisition there, only being beat out by a company that really was part of IBMs Smarter Energy Team at the time, and obviously direct fit into the renewable energy space.
3:03 Later on, Mario and I used to have lots of discussions around talent potential, M&A, and ultimately when he sold to UTC, not related but we actually do quite a bit of executive leadership work for UTC, both at the Chief Digital Officer and the Chief Information Security Officer, so touching all of those checkboxes together. So, with that, welcome Mario. It’s really great to have you on this podcast, and of course based on a deep relationship that we have. Let’s start off, tell me a little bit about yourself, and importantly your professional journey that brought you to where you’re at now.
Great, thank you Ken for inviting me to your podcast series, happy to be here, and yes, we do go back quite a bit. My personal background, I’m an Industrial & Systems Engineer, started my career consulting in the Oracle ecosystem, doing large scale European implementations, and in that journey, I was managing a master data management practice for Hitachi Consulting, and at that time a pipeline customer had a pretty significant pipeline failure. They said, ‘Hey, can you help us with this predictive analytics interest that we have in using our own data?’ I pitched the idea inside of Hitachi and they weren’t interested, I couldn’t sleep at night thinking about the opportunity, and that led me to start Predikto back in 2013. I did that until the acquisition about 18 months ago by UTC.
So, yeah, background both of consulting, a little bit of strategy work at McKinsey & Company, back into the consulting world, and then I made the transition of, well, how hard can it be to create a product centric start-up, instead of consulting? I was deeply humbled, a very-very different world, but fascinating experience that eventually led to the Exit Zone.
5:16 Excellent. We’ll take our questions in maybe a bit of a chronological order, because I think both your journey as a founder, an early pioneer and founder in this industrial analytics space at Predikto, there’s a number of questions I’d love to bring up relative to that experience. Of course, the exit to UTC and continued pioneering work you’ve done there. And then I also understand that you’re working on your next big thing relative to analytics as well, something instilled at this point. So, I’m as excited to hear as much as you’re willing to share on that. Starting with Predikto, tell me a bit about Predikto at the time, what was the company’s focus in the space?
Very quickly we realized that the large industrial asset-heavy industries were generating a lot of data, and weren’t really using much of it, and the pain related to reactive maintenance was a pretty easy and simple story to understand and pitch. So, if I can use your own data and tell you ahead of time that a bad thing is going to happen to this locomotive, or an aircraft engine, and you’re able to proactively repair it, how much value does that bring to you? And everybody was trying to improve in that area, and there were people dedicated inside the organization, and consulting companies that were trying to continuously improve in that area.
That world of maintenance predictive analytics is a heavy physics-based, rules engine-based world, where if the engine crosses this threshold of temperature for example, one variable, then notify. The idea of using more data, and specifically just advanced analytics machine learning type analytics was not widely used. So, the idea of, well let’s help create a product to accelerate the use of their own data and combine that data with NOAA and NASA weather in order to predict, that was the idea. It was a very simple sales pitch I guess you can say, very-very-very challenging to do, and very quickly we realized that highly customized consulting heavy type work, and that one day the scientist could probably do about five custom model trainings per year, using these advanced algorithms.
And when you have a fleet that has hundreds if not thousands of different custom failure modes, because of all of the different types of mechanical systems inside of these transportation-heavy equipment, you just don’t have enough data scientists in the planet to be able to go tackle them one at a time. So, very quickly Predikto pivoted to try to make the data scientist obsolete, which is a crazy idea, but for us that was the pitch. We quickly tried to automate the process of creating machine learning models, we were early in the Auto ML space without it being called Auto ML! We got about 85 percent there, and with Predikto instead of doing five models per year, we got to about 5,000 custom models a year with one person. So, that brought scale, that brought some very interesting large Fortune 100 type customers, and a lot of very interesting partners as well.
9:26 Why the focus on transportation, as you said, versus other domains at the time? And I’m thinking health of course was something a lot of people were talking about, pattern matching for x-rays as an example.
As a start-up you’re always running out of money, and you have incredibly limited resources. Early-on we landed CSX and Canadian Pacific, two of the five largest railroads in North America, we landed them very early, and by trying to focus on ensuring that the product was a success, we naturally started to enhance the platform in being able to be very good at big things that move over space and time. This thing is moving from point A to point B, its generating data through that journey, the data being generated through that journey in space and time is being connected with NASA and NOAA weather data, and these weather stations are fixed.
Then you’re also tying it to what has happened to this thing; when was the last time it had maintenance? Why did it have that maintenance? What components and parts does it have? And by being focused in these use cases of big things that move, it naturally resulted in transportation being an area where we would be getting in front of potential customers, and they would see the connection of, ‘Wait, I have a big thing that moves as well’, so rail with bullet trains, locomotives, commuter trains in Europe and London, was early use cases as well with Siemens.
We ended up doing some work in space, in being able to predict… can’t really talk about the customer due to NDAs, but we were able to predict a health condition in humans. The big thing that moves was the use case in this case, was a human that moves over space and time, being able to connect particular data from weather as well. Other companies were focused more in continuous manufacturing, or process manufacturing, and the data when you got really deep into it was slightly different. In our case we just had a very effective solution for transportation, and we didn’t start by saying let’s go after transportation, it’s just as we were landing customers, that’s the direction that we ended up focusing on.
12:18 Excellent, again we wish we would have invested, because the idea of focus and then moving to adjacencies, as Geoffrey Moore calls, ‘Jumping to the next lily-pad’, is exactly what we look for, and we invest in, in many of our founding companies.
Who were some of your key competitors at the time? Because you were really early into this space, especially into the sector.
Yes, very early, back in 2013, and I don’t think GE Predix was big, I think they really invested in marketing one or two years later. But early-on we were seeing IMB, P&Q, Yarsley, Clockwork, in the oil and gas space, obviously eventually C3 IoT with uptake, and GE Predix, there was this GE and Accenture merger, I think it was Solairus in the aviation space. Then naturally we would see a lot of early ideas, early start-ups that three or four years down the road they would lose some momentum. We would run into M-Tel quite a bit early-on in the transportation space, they were trying to move away from process manufacturing that I think was their focus. They landed a large transportation customer, they went after that space aggressively, and we would run into them regularly.
13:51 You’ve listed an awful lot of companies I know, especially the last one, M-Tel, I’ve got some good ex-Wonderware friends that were a part of that, in terms of really driving their process automation stuff.
14:02 The other one of course, because you’ve mentioned SpaceTime, which was SpaceTime Insight which Nokia eventually acquired. I can say that SpaceTime, you guys, and of course the company we finally selected Utopus Insights, there were really the three finalists in the work that we did, in terms of this, so, bi-side landscaping. Many of our readers will know that we published a white paper very specifically on the impressions we got out of doing this bi-side, we looked at 147 predictive analytics companies, both looking at rotating equipment as one-use case, and then energy demand response was the other, and ultimately selected the five and then of course helped acquire the one.
14:48 But Mario, for a company that was as lean as you guys were in terms of people, you guys really did punch well above your weight at the time, I can certainly tell you that several years after the fact now!
So, it sounds like you had a lot of great successes across the client base that you were both talking about, what led you to exit when you did to UTC, and why UTC?
I definitely think we were early. It was tough getting in the room, and pitching to potential customers right after IBM, GE, and others had presented, this 25-person start-up pitching to this Fortune 100 was very challenging. We got to be very-very good at delivering on time a 90 day pilot for a fixed fee, so that allowed us to go in laser-focused with a very concise pitch that said, ‘This is what we do really well, all of these other 99 things we do not do. And if your use cases and your pain fits really well with this capability that our technology does, this is how we would run a 90-day pilot.
So, in the case of UTC, we got in there through a partner that introduced us to Pratt & Whitney, and we were very effective in bringing significant value in the 90-day pilot. Actually, halfway through that pilot we expanded the work and went after a different use case as well, so we were doing parallel initiatives. They were very-very effective, and it was after that pilot whilst we were in conversations to expand the licensing agreement, the parent company UTC who had recently been investing significantly in a massive digital transformation, they came and they said, ‘Hey, we’re very interested’. We were actually running a process, and at the end we had multiple offers on the table, and it was a successful outcome for us and the investors.
17:16 And those that wish they would have invested!
We invest in it a lot in this industrial analytic space, and I know you may be emerging as doing something in the space again, but in terms of your peers, the other founders of industrial analytics start-up, if you had a chance to advise them, and you may be doing so already, what would you tell them in terms of your own learning and experience, having led Predikto and exited it?
It’s fascinating. I’ve become friends with some CEOs of direct competitors, because we would potentially be pitching at a similar event or being invited to opportunities and we would sometimes connect. We understood our similarities and our differences, and we were all trying to get something off the ground, which is incrediblychallenging, so that the shared scars are a bonding opportunity. I would say being able to deliver on a pilot incredibly well-organized on time, in a specific timeframe, for a specific flat fee, is a very effective way of getting in the door. It is very confusing in the data analytic space today, there are so many options from the large cloud platform players, AWS to Azure, to specific applications, to consulting companies who have some additional IP to help differentiate them. It is massively confusing.
So, being able to be very targeted to what makes you special and different. Being able to connect on their need, their use case, in a very-very targeted focused way. And being able to pitch and execute on a very specific pilot, I think the 90-day period is a nice amount of time that gives you the opportunity to add value, but its short enough as well. I think those were all things that helped us significantly, and I would say to not be afraid of significantly partnering with somebody.
Investors don’t like start-ups to attach themselves too strongly to a large partner, because they like you to have that freedom and flexibility. But in this space, I found those who did partner really well with somebody like an AWS or somebody else, the inflow of deals was a big advantage to acceleration. So, I would say don’t be afraid to do that early-on.
20:21 Great advice, and certainly I’ve heard the ‘common sense’ about maintaining and being able to play the field better. I agree with you, you do need to make an early selection of both partners, and as we advise may of our companies, know who your acquirer is going to be early-on. Make sure that you have the opportunity to show a ‘little leg’, as we like to say, along the way as well, which clearly you guys did.
Let’s transition to UTC and some of the work you did there as well, what was your role and remit at UTC?
UTC acquired us, we joined them as part of their digital transformation, UTC being the parent company with four business units, Otis, Carrier, Collins, and Pratt & Whitney, that’s going through a pretty significant change as well since Otis and Carrier are being spun off as independent companies, and they will announce Raytheon on merger with the remaining aero companies that’s happening this year as well.
So, at UTC I was primarily responsible for ensuring that the Predikto acquisition went well, went smoothly. UTC is an excellent well-run organization, lots of experience acquiring and merging with companies that have thousands of employees, in the case of us 20 people is a different story. But primarily responsible for scaling that team and ensuring the technology scaling inside of UTC with many use cases. So, that was my main responsibilities, but also obviously helping with the broader data and analytic strategy at UTC, and how the different business units and everybody is trying to get better and faster at using their own data with tools, and partners, in order to beat the competition.
22:26 I fully concur with you in terms of the organization of Vince Campisi particularly, as overall Chief Digital Officer, we’ve been very impressed watching him in terms of the decisions he’s made, and of course with help of some of his hires as well. But even as the organizations are splitting apart, we see that excellence going with the various units. As many of you know we interviewed Carrier’s CISO, Chief Information Security Officer, not that long ago on a webinar that we did on industrial security, just judging by the quality there, hopefully many of our listeners got a sense for the quality of the leadership in that organization.
23:12 Can you give me a sense of some of the high-level use cases that you worked on. I’m assuming rotating equipment generally would have been one of those, but where you saw in some sense the most take-up in the organization.
Obviously we were already working with Pratt & Whitney before the acquisition, and that was a heavy focus for us, and just helping to improve the reliability in the aftermarket services of the engine health. So, being able to focus on predicting when an event would happen, and the very complex and highly sophisticated relationships that Pratt has with their customers.
Aviation is a pretty complex space, you have the airlines, and then you have the manufacturers, the Boeings of the world, then you have Pratt as the engine supplier to that. So, there’s multiple layers of customers, and multiple layers of people involved in being able to take action. So, if you have an algorithm, a classification machine running algorithm that’s able to predict a failure on an engine, a very specific failure type, it’s complicated in order to go through the multiple layers to eventually turn that into an action. Once you’re able to do that, the achieved value is massive in the aviation space, so very-very proud of what the team is doing.
24:49 You mentioned, ‘In the aviation space’, how did you benchmark yourself relative to your peers in the industry, or even in adjacent industries. How do you know UTC at the end of the day truly was a leader in what you were doing, or who did you look for in terms of sometimes emulating your learning from?
We actually got started in the aviation space with FedEx maybe halfway through our journey. We went into the FedEx use case, not having any idea about aviation, we didn’t have anybody in the team that was an aviation expert, we didn’t even know what an AOG was, which for the industry is an aircraft on the ground, which is aviation one-on-one for people in the space. Very quickly we realized the value that we could bring to the table with a true positive, usually has an extra zero or two to it, compared to other use cases, so, that makes the ROI conversation much easier.
But in the sense of how to benchmark ourselves, or trying to compare how effective we were, we just had this very specific platform for big things that move. So the ability to go into a customer and take their data about an aircraft, and let’s just say 500 engines over a year, and how those engines are being swapped on a tail on an airplane, and following the journey of an engine and its flights, and all of its sensor data, and how the data is connected to NASA NOAA weather data; being able to do an ETL job of that magnitude, of that complexity in 48 hours, we were just crushing the competition, and being able to stitch the data together so quickly, to then apply what we called max, the Predikto machine learning automation engine, being able to apply that and create hundreds of custom models in a matter of weeks, and delivering a fully functioning application, nobody was able to do that in that space.
So, that speed in bringing very complex machine learning analytics to industries that were already verysophisticated, using data analytics, that was a big differentiator. We did about 36 different pilots and deployments of our technology, and we competed against IBM, maybe 12 or 13 times, and we never came in second, as an example. We loved those competitive pilots where the data was shared with multiple parties, because we knew we had something that was very specific, and we qualified our pilots and customers incredibly aggressively. It was too expensive for us not be successful on a pilot, so we were very specific on ensuring that the data that they had, the use case they had, the problem that they had, was a perfect fit for our technology so that we would be successful.
28:28 Given that you’ve had the unique opportunity to take a young very pioneering disruptive company and set of technologies into a large industrial, generally what do you see as the key challenges? I know technology pushed in many of these cases, it’s always early on the Gartner hype curve if you will, but the reality of hitting an organization where they’ve got ‘The Adams are meeting the Bensons’, in some sense, is where you really get to see the true take-up if you will of technology. Where did you see the largest challenges, not necessarily just in UTC, but generally among the industry?
Yes, it is a very complex industry, these are large organizations usually over 100 years old, they’re very good at what they do, and they’ve got to that scale very profitable industries and companies, and they already invest significantly in data analytics. So, you need to make sure you understand where you fit in that ecosystem, in a highly competitive ecosystem, and why you.
One early lesson, and frankly people need to look at themselves in the mirror and say, am I a tool that enables my customer to use the tool, for my customer to get the glory and solve their problem on their own? Or, am I a SaaS go-to market type of company, where the combination of my people and the tools that I have solve the problem for my customer?’ We were encouraged to be a SaaS company in an early space where our customers didn’t really know how to buy SaaS, and our customers don’t want a small start-up that is in SaaS go-to market, to be the one solving the problem, they want to be able to buy the tool and solve the problem on their own. So, those were early lessons of, ‘How does my customer want to use my capabilities’, so making sure your go-to market is well aligned with that.
So that’s one area I would say is challenging, and the other is advanced analytics is completely meaningless if you can’t have the human perform the action at the end. So, it doesn’t matter how amazing the software looks, how fast you can process the data and ingest it. How many analytics you can do, or how accurate they are, if in the end it dies at the PowerPoint, and it says, ‘We could have done x, y, and z, with this model’, but you never did. That is what’s preventing value from being delivered in these heavy industries. There are a lot of highly complex internal systems, processes, and walls, that are making digital transformations of large industrials highly challenging. It could be the business model, it could be the relationship that your customer has with their customer, I mentioned aviation as an example as well as other industries.
There’s just multiple layers of customers and contracts that say how data can and cannot be used, or who has the incentive of acting or not acting, because there are after-market contracts, that dictates who pays for the failure expenses of an early failure of a part, and maybe your customers are incentivized to not act because somebody else is paying for the problem. So, all of those are very-very complicated realities.
We were working with a bullet train company and we were able to predict that they were going to have a failure in a bullet train that day, and they would look at us and say, ‘I don’t have any spares. I cannot do anything about it, I have to send the train out anyway, thanks for letting me know’. So, your kind of staring at this, we were successful but the environment, the system, the structure prevents that value from being achieved, so it’s very-very complicated.
33:12 One might even say that could be the underlying bi line if you will for digital transformation! The technology is the catalyst and it’s an enabler, but it still has to basically accelerate existing business models, existing partner relationships, financial models and everything else. Thus, the conundrum of the two meeting, and where a lot of the challenge tends to be in digital transformation projects.
33:39 Let’s talk a little bit about what’s next. Given that you so aptly named the company Predikto, I’m going to ask you to predict where do you think this space of industrial analytics is going? And we call it generally Digital Industry, to refer to everything from the data collection, all the way up to responsive on the data insights, where do you see things going over the next several years?
I think naturally continued investments in different technologies, to continue to move the ball forward. I think we’re just moving it one or two yards at a time, it’s painful and it’s very expensive. Those who are able to operationalize digital technologies to accelerate what they’re doing, are going to see a massive acceleration, and start to see the 10 and 20-yard passes. So, those organizations who can get there faster are going to start to see a massive difference in how they’re able to differentiate themselves from their competitors, by either reducing cost, or being able to take advantage of new revenues.
The challenge is that you’re dealing with organizations that have over 10 billion dollars in revenue, in order to have a significant impact on the bottom line, it takes a lot. Being able to improve something by .1% is not as interesting. But having said that, there’s a lot of fear out there that my competitors are going to be able to use digital technologies to improve things faster, and that one or two-year sprint ahead of me is going to have a significant difference. So, obviously continued investments, I see that happening. I think a lot of people are starting to realize how they need to be working with start-ups, it’s crazy to have to wait six to nine months to be able to get a basic statement of work through a legal procurement. So, a lot of organizations are starting to say, ‘Well how can I get that down to three weeks, or one week?’ ‘How can I do 30 pilots with digital technologies this year, instead of two?’
So, those who are looking to accelerate just getting their hands dirty and engaging with different start-ups and other organizations in a fast and effective way, that’s a trend that I’m seeing. I like the idea that many organizations who have created innovation labs, or innovation centers, are realizing, ‘It is difficult for us to behave like a start-up’. There are too many antibodies inside of the mothership that make it challenging, let’s try to invest and partner with organizations who can help us create an independent start-up, where we are maybe side investors, or we are providing some subject matter expertise.
I’m seeing some venture studios work with large industrials very effectively, that’s a very early trend that I’m seeing. But I’m starting to notice that large industrials are saying, ‘Hey, I am struggling to do this fast, maybe I have to create a separate entity’, and give them the freedom to operate outside of the four walls of the large incumbent, to be able to accelerate innovation. Because the traditional R&D way of doing innovation was effective 30 years ago, today times are changing. There’s a lot of new companies coming to the market, and there’s a lot of fear that they will disrupt incumbent’s business models.
37:53 So, are you hinting to us about what’s next for Mario?
[Laughs] Well, as you said, I do have something in self mode in the analytic space, something I experienced personally, so working on that. My scars are of significant interest to a lot of big and small organizations, so having a lot of conversations on what’s to come. But yes, certainly dropped a lot of hints in there, and I’ll let you guys know how things are going in a few months.
38:28 Excellent, excellent. I don’t want to say that I missed out on this once again, so sooner the better. So. with that in mind, and on behalf of my ventures brethren and sisters if you will, what start-ups are the ones to watch in this space? I’m thinking particularly the digital industry space.
I like the start-ups that are very focused, Sight Machine is doing a nice job in the process and manufacturing space. There are a few that are gaining traction, smaller ones in oil and gas, or utility space to Metis based in the UK and US as well, is another that I’m liking. But anybody who is significantly focused and that has a lot of stickiness with their customers, is somebody to watch. A lot of those are realizing that the sale cycle’s been so long they have to be more of a hybrid, and not just be a pure software licensing type of play. They’re mixing software with consulting to be able to fund their ideas, their growth, and their IP, development as well.
But yeah, there’s a lot of companies out there, and I’m also seeing the big players like the AWS’s, the Azures, and the Google’s, expanding, continuously expanding their platforms to be able to erode and eat into the pure analytics software company’s product strategies as well. So, lots of noise out there, I was at the Gartner Data & Analytics conference last year, and my god it is incredibly busy with so many companies going after this space. So, anybody who is really focused in your industry, your type of use cases, those are companies to watch.
40:34 Excellent. And the final question we always like to do on our podcasts, any recommendations of books or other resources you’d like to share, whether they’re related to our conversation, or life in general?
Two books that I recommend regularly, I would bring them up here on this podcast. The first one is called, ‘The Mindset’ by Carol Dweck, it was written maybe 20 years ago, and in the last 5 years it has gained a lot of momentum. Carol Dweck does a fascinating job, and it’s a quick read, she does a fascinating job of trying to split humans into two types, there’s the growth minded, and fixed minded. I found that book fascinating because it helped me understand how people think, and how they might react to certain situation. A growth minded person is somebody who is constantly trying to learn, mistakes aren’t that negative or critical, it’s part of the learning process for them. A fixed minded person is very good at one thing, they stick to that one thing, the worst thing that can happen to them is to make a mistake, so they’re very cautious.
That helped me a lot in understanding my team and different personalities. It also helped me understand partners, potential customers, and people I was interacting with on a daily basis, and even with my own family, differences, and children. So, I highly recommend that book, ‘Mindset’ by Carol Dweck.
And another one that I used a lot and helped to shape the way I would run my team is, ‘Traction: The Entrepreneur’s Operating System’, by Gino Wickman. It’s kind of like an agile for management, it helped me bring a lot of process improvements, how to evaluate my team, the people analyzer and how to do 3600 evaluations on the values of the company, and if the people get it, want it, and have the ability to do the job. Also, how to stay focused, what do we look like in three years, and in one year, and in order to execute on that vision, what do we need to do this quarter, and let’s stick to that. We tied it with OKR, I think that was developed by Intel or Google. So, highly recommend that book Traction for any leader of a large team, or maybe a startup founder, who is looking to bring some sanity into how they’re running their teams.
43:28 Both great examples of books. I like the growth versus fixed, I always hear it as builders versus operators, in terms of the Irish-type setup there.
It’s been my distinct pleasure to interview Mario Montag, somebody who we have a deep relationship with at Momenta, CEO and Co-founder of Predikto, VP and Chief Data & Analytics Officer at United Technologies, and soon to be successful entrepreneur yet again. So, we’re very excited to have featured you on this podcast, and looking forward to continuing the relationship, and the conversations as well.
44:06 So, thank you very much Mario, and to our listening base, have a great day. Thank you very much.
Thank you, take care.
[End]