Dr. Matthew Carr
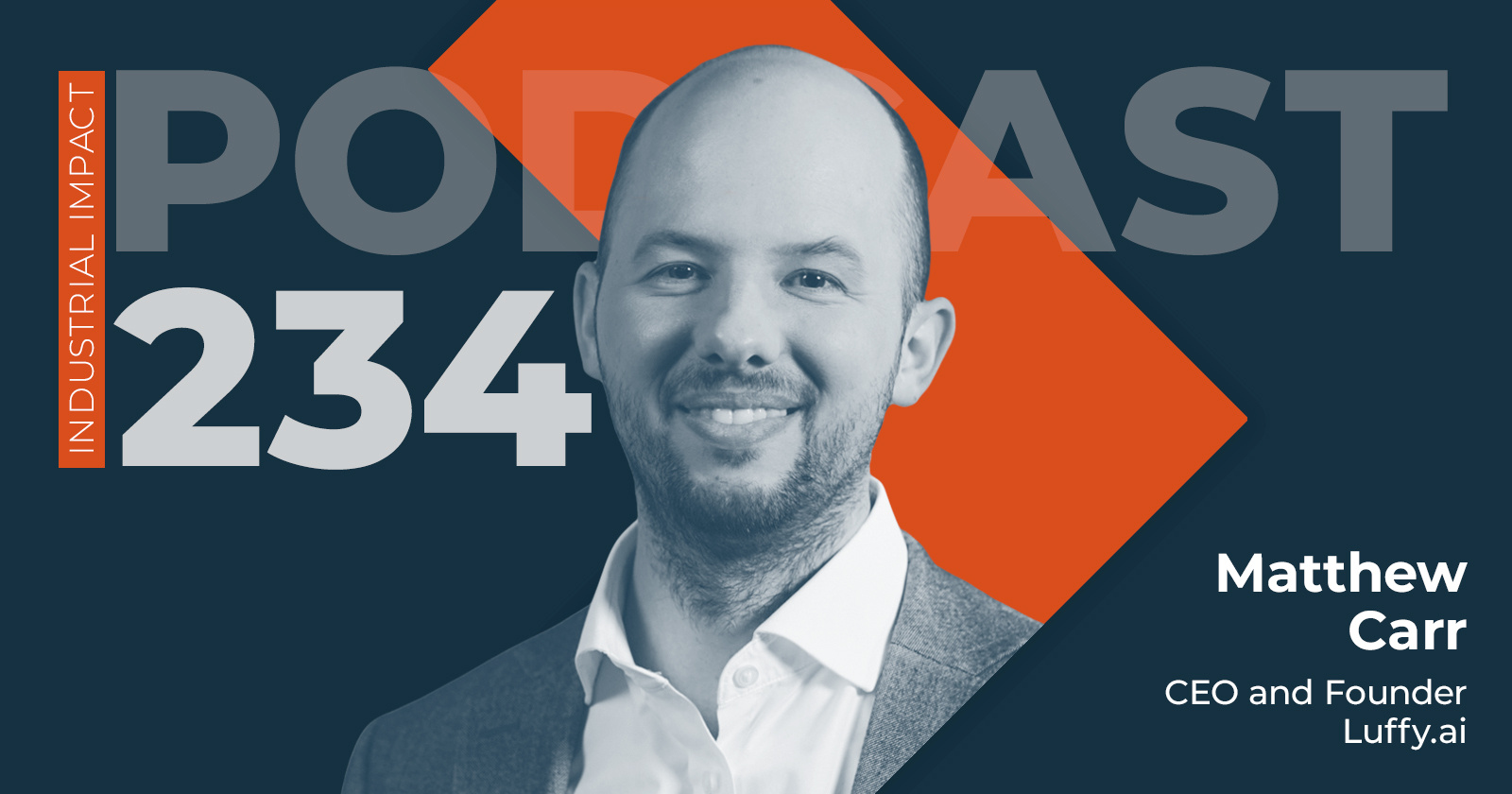
TRANSCRIPT
Ken: Good day, and welcome to episode 234 of our Momenta Industrial Impact Podcast. Today, I'm pleased to host Dr. Matthew Carr, CEO and co-founder of Luffy AI, the first adaptive AI platform for industrial optimization. Momenta led the recent funding round for Luffy, our 55th portfolio company. Matthew is an experienced data scientist and entrepreneur who has worked across AI, data science, fusion physics, and scientific research. Before founding Luffy with Alex Meakins in 2019, Matthew worked as a fusion physicist at the UK Atomic Energy Authority. At UKAEA, he developed fusion reactor simulation models and conducted novel research into Bayesian inference algorithms for estimating the reactor state and performance. Matthew earned a Doctor of Philosophy degree in Plasma Physics and Nuclear Fusion Technology from the University of Sydney in 2013 after completing a Bachelor of Science in Physics at UNSW. He is an expert in data science, neural networks, deep learning, simulation, and modeling. Matthew, welcome to our Industrial Impact podcast.
[00:01:49] Matthew: Thanks, Ken. It's a pleasure to be on your podcast.
[00:01:52] Ken: It is a pleasure to have you; what a great bio leading into this. You and I know it took me more than one tries to get some of these terms correctly, but it should be a fascinating podcast and funding we've done most recently. We call this the Digital Thread podcast, which is about one's digital thread regarding the defining interests and experiences that brought them to this point in life. What would you consider to be your digital thread?
[00:02:19] Matthew: That's a fantastic question. I've always been passionate about industrial technologies and power generation. I've also been very passionate about the energy transition and seeking out technologies I can work with that push towards decarbonization and Industry 4.0 and even Industry 5.0 levels of automation. It was always a pretty even mix of curiosity for physical systems and the data science algorithms that might enable new types of operation and efficiency. In my undergraduate degree, I initially studied electrical engineering and spent some time around solar cell manufacturing facilities and wind turbine research. I then transferred to physics and mathematics to go deeper into the processes and algorithms that control them. Then, ultimately, I moved into fusion physics for my PhD studies because I saw it as a fantastic way to combine my passions for advanced engineering, physics, and data science. It was during my PhD that I ended up discovering startups by accident. A former lab graduate had founded a startup with my PhD supervisor; they were trying to make a new electric radiation source for producing medical isotopes and detecting contraband at airports. It was just a fantastic challenge. I was initially given a part-time job as a scientist there but ended up running that small startup for two years. When I started, it was just a benchtop experiment. It just looked like a lab, a benchtop lab exercise. By the time I finished there, it looked like a product. We had it in a travel bag that looked like a ski bag, and you could carry it around and easily demonstrate it. The nice thing about it was it's a radiation source you could turn on and off.
From that experience, I just fell in love with startups because of the off-the-wall innovation and creativity, the challenge of what you're trying to do with the disruption, and also sometimes even just the chaos of needing to solve problems quickly and efficiently because you don't have those luxuries like you might have in a more prominent company. That seeded my interest in entrepreneurialism and combining that with deep tech research and ideas. From there, I moved on to my postdoc at the UK Atomic Energy Authority, where I was working at the world's premier fusion research lab in the Culham Science Centre. There, they have JET, the world's largest fusion reactor, which burns at the hottest temperature on this side of the Milky Way, which was a fantastic opportunity. I would venture to say that fusion reactors are the most complex machines ever developed. They have tens of thousands of sensors and hundreds of actuators, and I was working on simulating the reactors and trying to infer their states with the eventual goal of optimally controlling them for the highest levels of fusion efficiency. It was at UKAEA that I developed my understanding of the need for these new types of data science and modeling and AI tools so that we could better infer the state of engineering machines and how we can control them, which ultimately led to forming Luffy as a team spin-out from the lab.
[00:05:11] Ken: You know, I'm always a big fan of cross-domain thinking, and in your case, I'd also tie in convergence because of how these fields have come together to help you found Luffy. You made quite a broad jump from nuclear fusion to what I'll call industrial AI, which, of course, is our investment thesis. What attracted you to industrial AI in the end?
[00:05:36] Matthew: That's a great question. It felt like a natural progression because of our experience modeling and controlling fusion reactors. We saw that the advanced simulation, modeling, and control systems needed for fusion could apply similarly to manufacturing robotics. But what indeed attracted me was the potential for massive impact. Imagine a future where manufacturing, the backbone of our society, is revitalized. Rising labor and energy costs have caused many industries to decline or migrate to countries with cheaper labor. This trend is concerning, but AI could help reverse it. AI can increase automation levels in factories, make processes more energy-efficient, and incorporate more recycled materials, promoting a circular economy. Innovations in industrial AI could allow us to bring back a lot of manufacturing that has left and make it competitive again. McKinsey estimates that nearly $1 trillion in value creation is possible in manufacturing if we can fully develop and realize these technologies. It will create new high-tech jobs and enrich our economies. This is the future we envision with industrial AI, a future full of hope and optimism.
[00:06:58] Ken: You've done a better and more eloquent job explaining the Momenta investment thesis than we've ever done on our website. I will have to get the transcript of this. Essentially, it's the same trend, right? Reshoring, labor shortages, and looking at leapfrog technologies. Over the last 18 months, AI has emerged as the leading leapfrog technology. It's a great time to start a company like Luffy AI. Tell us about Luffy's origin story.
[00:07:39] Matthew: I met my co-founder, Alex Meakins, on my first day at UKAEA. We shared an office and quickly bonded over our interest in deep physics problems, data science, AI, and fusion and robotics manufacturing challenges. From 2013 to 2015, during our early collaboration, the profound learning revolution led by DeepMind was in full swing, and it was hard not to hear about it constantly. What fascinated me was the varying levels of maturity and adoption across different fields. Supervised learning technologies for image and speech recognition were reaching commercialization and maturity, but technologies for control, like deep reinforcement learning, were still in basic research. In factories adopting AI, you'll often see cameras for defect detection and cloud-based dashboards for predictive maintenance. Still, the control systems usually rely on traditional technologies like PID or MPC. This is because supervised learning techniques are data-hungry, requiring vast datasets that are expensive and often biased, making it hard to train a general control solution.
Meanwhile, research in deep reinforcement learning, which involves training based on simulation, often fails to transfer accurately to the real world. Alex's PhD research in evolutionary AI techniques showed that biological approaches could create adaptive AI systems, freeing up the constraints of traditional AI. After years of discussing and researching algorithms, we believed this was the key to solving these problems. We formed a spin-out team from UKAEA in late 2019 to develop an adaptive AI platform technology with this goal in mind.
[00:09:59] Ken: When discussing the unique technology underlying Luffy AI, you mentioned biologically inspired techniques such as neuroplasticity and neuromodulation to create neural networks that can truly learn and adapt at the Edge. How does this adaptive intelligence, or adaptive AI, as you referred to it a moment ago, differ from traditional forms of industrial optimization or control?
[00:10:23] Matthew: The most widely used control systems today are PID controllers, which are essentially a tuned set of mathematical equations. You have to tune them for each system you deploy them on. They may work perfectly initially but are only as good as their calibration. If there are any changes in the system, like wear and tear, component failures, or even variations in feedstock materials, it can move away from the optimal tuning region. This leads to degraded performance, ultimately wasting energy and producing more variable product quality. Another more advanced class of controllers is MPCs or model predictive controllers, which use physics models to simulate the system at each time step and determine the optimal control actions. These systems work well in processes with suitable time dynamics and accurate physics models. Unfortunately, accurate models are unavailable for many processes, the dynamics are too complex, or maintaining and operating MPC systems is too expensive. The majority of the industry still relies on simple PID mathematically-based controllers susceptible to tuning issues.
Adaptive AI overcomes these problems by enabling the creation of a new class of neural networks that can learn and adapt at the Edge. It employs biologically inspired mechanisms, such as neuroplasticity and neuromodulation, embedded in the neural network, using real-time feedback from the systems to tune themselves to the machine in question. It uniquely tunes itself to that machine, embedding an optimization loop into the neural network that can adjust based on live data. This addresses the problems in supervised learning, where networks are usually static after training. In many supervised learning systems, manufacturers train on large datasets. Still, operations change quickly, causing the system to drift out of the dataset, necessitating retraining in the Cloud and leading to performance gaps. The real advantage of adaptive AI technology, like what Luffy is developing, is that we can start training on digital twins of the process that don't need to be precise models but need to account for the main variances. These are usually digital twins or simulation models that manufacturers or operators already have. When trained on these variances, the neural network can tune, adapt, and learn the system at the Edge.
[00:12:48] Ken: To demonstrate the power of your solution, your technology already spans industrial processes, robotics, and even drones. What is the standard solution thread in these different domains?
[00:13:06] Matthew: We currently test the system across various application domains. What we see in common is that these are all dynamic, non-linear control problems. These are processes that can change quickly, or the value of the outputs is susceptible to how it's controlled. Customers often face challenges due to the limitations of PID control systems. These systems work but only when correctly tuned. Changes in the system, business goals, rising energy costs, or increased use of recycled materials push the control system to its limits. These customers, like us, believe that AI control could solve these problems and enable new operating modes or access the full potential of their hardware, which they currently can't achieve.
[00:13:52] Ken: As another form of demonstration, what have been some of your early wins?
[00:13:57] Matthew: One of our first manufacturing customers was a UK-based OEM equipment manufacturer producing high-end aerospace and automotive composite parts. The process involves a mold tool where composite materials and resins are laid, followed by a heating and cooling process to cure the parts. Because these are high-value parts made in batches of only 100 at a time, the quality is susceptible to how the machine is run. They had a traditional PID-based tuning process that allowed them to tune it each time the part or mold changed. This method could achieve good results but was labor-intensive and required expert users. They wanted an AI-based system enabling any user to achieve expert-level results. An apprentice or new user could operate the equipment and produce significant parts without needing three or four years of experience. Our AI control system can self-optimize for variations in mold tooling, materials, and part demands. When we developed and tested this AI control system in their manufacturing line, we immediately saw a 10% improvement in energy efficiency. We also observed a significant improvement in the temperature span across the device surface. The real achievement was that this was just out of the box. The AI was self-tuning and self-learning on the fly. We are now commercializing this system with them, and based on this trial, we have signed new blue-chip customers, which I am excited about, although I can't announce them just yet. I hope to have some exciting developments over the rest of the year.
[00:15:46] Ken: Well, I know you've got a significant pipeline of opportunities. I'm curious, though. Using an old analogy, I'm sure people don't go through the phone book looking for advanced AI or autonomous solutions, or Luffy, for that matter. How do your clients ultimately find you? More importantly, how do you engage with them to solve these industrial AI challenges?
[00:16:07] Matthew: That's a great question. Many of these companies have already learned from experience that they need to adopt AI technologies. However, they often encounter professional services or highly customized solutions. The big differentiator for Luffy is that we offer a platform technology. We have a growing product library for a range of industrial unit processes. When customers come across us, it's easiest if they already have a process in our product library. We can then export this AI controller, which can self-tune and self-adapt to their process equipment, enabling them to adopt and scale the technology. If they have a new process we haven't worked with before; we typically work with them using a digital twin they already have. We run a general training process on these digital twins. Because of the adaptive capabilities of our networks, the solution reliably transfers to the real world. It can easily transition from one piece of hardware to the next of the same class.
[00:17:14] Ken: It sounds like you've already implemented some best practices in terms of end users and usage. How do you know when an organization is ready to adopt your solution? What best practices have you seen in realizing that potential value?
[00:17:30] Matthew: That's a great question, Ken. A common factor we see is a high level of digital maturity. Organizations that have invested in sensing and digitalization following the Internet of Things revolution have a better understanding of their plants. However, this sensing often can't be integrated into older PID and MPC systems for control, leading them to seek more advanced AI-based systems. They may already have control systems and digital twins and have tried supervised learning techniques but found them limited. It's important to note that we don't need advanced data science teams to work with our technology. We can work with the engineering models and control engineers already in the plant, making it easy to meet them where they are and build an AI solution.
Another best practice is taking a holistic view of the process. It's essential to consider the operators and the people running the machines. Making the system user-friendly and empowering operators is critical to driving AI adoption in this space.
[00:19:02] Ken: Now, would you say AI adoption in this space—Momenta has been investing in industrial optimization for over a decade, but we've often noted that few systems are genuinely used in an autonomous, closed-loop application mode. You mentioned PID is always a closed loop, right? MPC is essentially a closed loop. But it's often operator recommendations rather than a close loop regarding AI. Are you seeing that change? What are some key motivations for your clients who are now considering closing the loop?
[00:19:38] Matthew: It's a combination of economic and societal changes beyond just technology. The Internet of Things boom led to significant investment in digitalization, but many factories haven't been able to utilize this data fully. Additionally, increasing economic pressure to improve efficiency and competitiveness against cheaper labor economies drives manufacturers to innovate and push their process limits. There's also growing societal pressure to decarbonize and reduce emissions, which is becoming more prominent in manufacturing. Moreover, there are pressures to adopt Industry 4.0 practices, switch product grades easily, use more recycled materials, and adapt to market conditions. These factors drive interest and investment in advanced control systems, as traditional methods can't meet these new demands.
[00:20:50] Ken: One of the companies we've worked with talks about a general trend of automation to autonomy, and what we've seen in those two- you can call them extremes of maturity is it's more like automation to augmentation to agility to autonomy because you stop in points along the way. It's interesting when you start to talk about agility and how important that plays into it, and that's what you're speaking to in this regard. From an Industry 5.0 perspective, certainly, augmentation as well, i.e., considering all aspects, all stakeholders in your process, especially the operators, and in some sense, the environment. Look, this has been a great conversation as we wrap up. I'm always curious about how you maintain your Edge as a leader. Any recommendations you'd like to highlight for the audience at this point?
[00:21:35] Matthew: One of the first books I would recommend is probably not a common one, but it's called "Value First, Then Price." It was written about value-based pricing, but I found it influential because it gets you thinking about value creation for your end customer. It makes you laser-focused on understanding how this person will benefit from your actions and how to make them quantitative. What benefits will they get in terms of economic or energy efficiency, decarbonization, or whatever it may be? How can I focus on making that clear? Once you can clarify the value creation, everything else will flow naturally. It also helps with your selling and almost makes the return on investment a no-brainer. I found it very helpful in making me more laser-focused on assessing which processes we can benefit most and where the value creation will be. More generally, learning from other people's entrepreneurial stories is beneficial. I love biographies, such as the Jobs biography by Isaacson or ones about Jack Ma from Alibaba, Elon Musk, and others. Reading other people's entrepreneurial stories is always interesting because you learn about their struggles and the mentality and commitment it takes to be a successful entrepreneur. Beyond that, I'd recommend some classic thought leadership books for startups. "Crossing the Chasm," for example, is a classic.
[00:23:17] Ken: What impresses me, Matthew, and this goes back to the very beginning of our discussion, is that for someone who, by all definitions, should be at the epitome of deep tech—nuclear physicist, Ph.D.—you gave very well-rounded recommendations about business and how to apply that technology to a better purpose. It probably says a lot about how well-rounded you are and is perhaps one of the key reasons, beyond the superb technology, that we invested in Luffy AI.
[00:23:42] Matthew: Thanks, Ken. That's a great compliment.
[00:23:39] Ken: Well-deserved. Matthew, thank you for your time and these great insights today.
[00:23:45] Matthew: Thank you, Ken. It's been a pleasure.
[00:24:17]Ken: This has been Dr. Matthew Carr, CEO and co-founder of Luffy AI. Thank you for listening, and please join us for the next episode of our Industrial Impact podcast. We wish you an impactful day. You've been listening to the Momenta Digital Thread podcast series. We hope you've enjoyed the discussion, and as always, we welcome your comments and suggestions. Please check our website at momenta.one for archived versions of podcasts, as well as resources to help with your digital industry journey. Thank you for listening.
[The End]
Connect with Dr. Matthew Carr on LinkedIn!
Book recommendations
Matthew recommends several books that have influenced his leadership approach. One of his top picks is "Value First, Then Price," by Andreas Hinterhuber, which has significantly shaped his thinking about value creation for customers despite its focus on value-based pricing. This book emphasizes understanding and quantifying customer benefits, such as economic or energy efficiency and decarbonization, clarifying the value proposition, and enhancing the return on investment.
Matthew also enjoys reading biographies of successful entrepreneurs, like those of Steve Jobs, Jack Ma, and Elon Musk. These stories provide valuable lessons on the struggles, mentality, and commitment required to succeed in entrepreneurship. Additionally, he recommends classic thought leadership books for startups, such as "Crossing the Chasm" by Geoffrey A. Moore, which offers essential insights for navigating the early stages of a startup.
About Luff AI
Luffy AI creates adaptive neural networks that allow control and adaptation at the edge. Their AI control systems can learn the nuances of the process being controlled and adapt to changes over time to maintain optimal process efficiency. For more information, please visit http://www.luffy.ai.