Martin Cloake
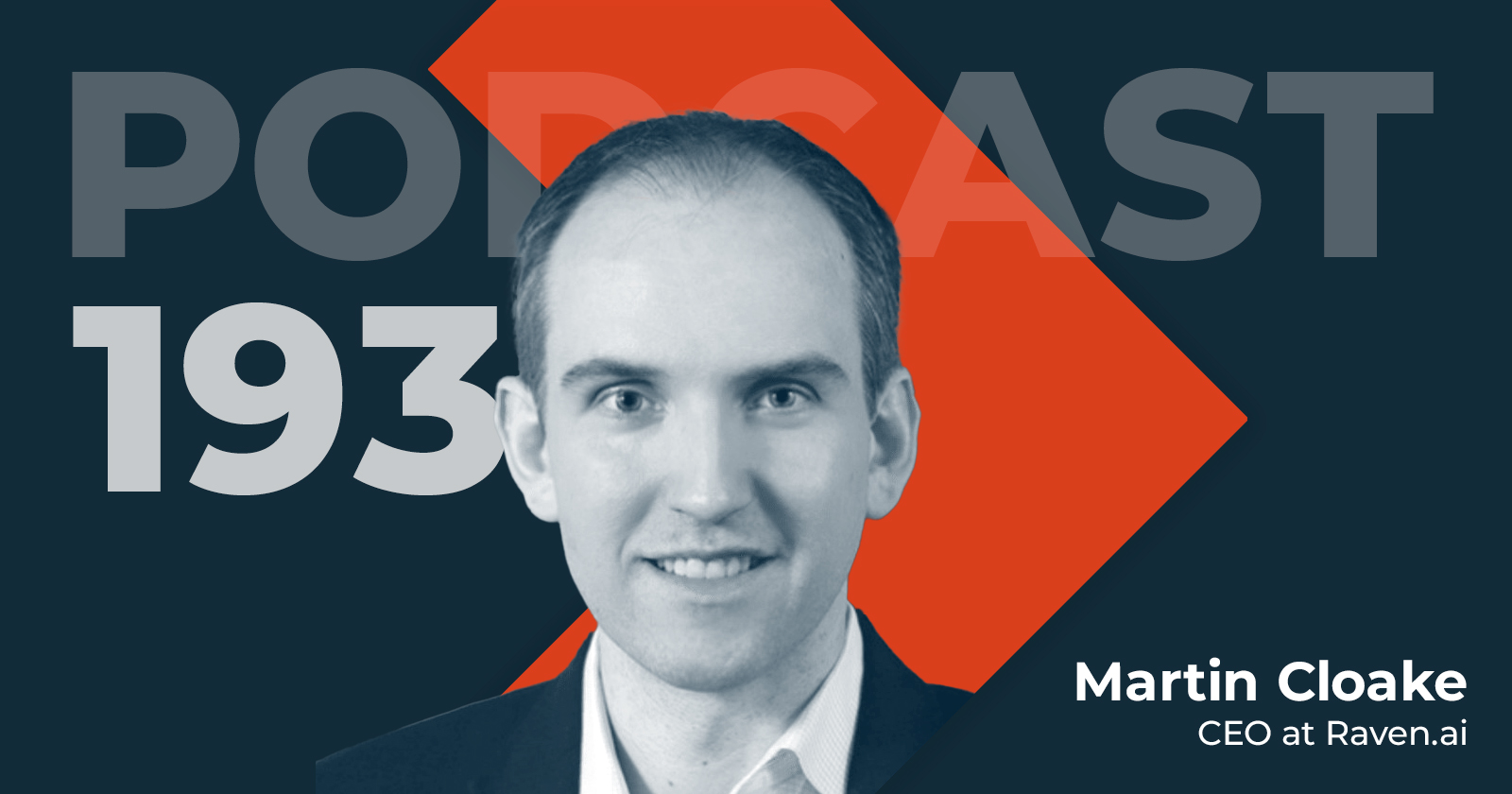
TRANSCRIPT
Ken: This is Ken Forster, Executive Director at Momenta. Welcome to our Digital Thread podcast produced by, for, and about digital industry leaders. In this series of conversations, we capture insights from the best and brightest minds in the digital industry- their executives, entrepreneurs, advisors, and other thought leaders. What they have in common is like our team at Momenta; they are deep industry operators. We hope you find these podcasts informative, and as always, we welcome your comments and suggestions. Good day, and welcome to episode 193 of our Momenta Digital Thread podcast series. Today I'm pleased to host Martin Cloake, CEO of Raven AI, the automated contextualization and OEE software for manufacturers. Raven is Momenta's most recent venture capital investment. Martin has over 20 years of industry leadership roles across manufacturing, data science, intellectual property, and operations management. He's an award-winning technology entrepreneur, holds multiple patents, and prolific writer on topics such as Industry 4.0 leadership, manufacturing, and augmented management. He has a Bachelor's in Mechanical Engineering from McGill University in Montreal, Quebec. Martin, welcome to our Digital Thread podcast.
[00:01:27]
Martin: Thanks for having me.
[00:01:28]
Ken: Oh yeah, good to have you. It was interesting; I remember one of the earliest conversations we had before we "discovered" Raven from an investment perspective. We had been trading jibes because it seems like you've got a popular community and podcast you do as well. It was fun to get to know you quickly in that vein. Of course, subsequently, we realized that you were sitting on a hot company, so we'll probably talk a little bit about both today. But you know this is the Digital Thread podcast, so I always like to start talking about one's digital thread. In other words, one or more thematic threads that define their digital industry journey. What would you consider to be your digital thread?
[00:02:08]
Martin: I think all you described- what has made this stuff interesting for me in general. I think one of the things that have always been part of my nature was constantly questioning to the point early on in my life it was just infuriating to have anybody around me. As I evolved through my education, I reached the point where science and math gave me the most satisfying answers to those questions, so I went down the engineering path. As I got into engineering, things started to seem clear about what I was interested in and how I would be able to do things in the workforce once graduating. Then, I got recruited by a manufacturing company right out of university. Then when I hit the shop floor of a manufacturing plant, my world changed somewhat because my view of how things worked in the workforce in the real world was somewhat skewed. What I had expected manufacturing to be based on my degree at McGill- a bunch of machines and data ready to be optimized by enthusiastic new grad engineers wasn't the reality of what I experienced. What I experienced on the shop floor was that the data available from these systems only scratches the surface of what's happening on the shop floor of manufacturing plants. If you want to drive improvement, which is what everybody ultimately wants to do- engineers, managers- otherwise, you need to identify and solve problems that are only evident if you walk around and meet with people and see things with your own eyes. Here's this realization that data people think paints the full picture is only scratching the surface. That opened my eyes early on in my career. Recognizing that to get value from data, you need to spend a lot more time thinking about human and people's roles in these systems, which isn't evident as a technologist. I'd love to dive into what I'm most passionate about, which is how to use data and how to use it to augment what people can do. It's not replaced; it augments. That's my passion; that combination of data, guidance, and human ingenuity, mashing it all together to make it possible for people to perform at levels never thought possible.
[00:04:10]
Ken: That's a very rich digital thread, and what I love about that is the, as you say, mashup of those very specific domains and backgrounds. As I mentioned, we led your Series A investment round at Raven AI. One of our strong theses is favoring those with deep industry expertise, i.e., the founders. I must say, you and your team brought that in, in spades. I know you spent about 15 years in the industry before starting Raven in 2015. You might have already spoken a little bit, but what were the top three insights you gleaned from this time relative to IoT?
[00:04:48]
Martin: Well, that first one, I alluded to it in my- in what I just described there, but the fact that data from digital systems, whether it be data from your PLC or machines, even from ERP in manufacturing, the data that you use to describe your performance doesn't describe why you are performing one way or another. I think that realization was one of the key things here where the data from these IoT systems is hugely valuable. Still, it doesn't explain why things are happening for you to understand the root cause of your efficiency or lack thereof. The first thing is this concept that there is currently blocked information on the shop floor that's in the minds of the folks working at the machines, that unless you find a way to tap it, you're never going to understand the problem enough to solve it. That's a key thing that likely encompasses several aspects of the problem we're discussing today. The second one is that when thinking about how to get value from these digital systems, it takes for granted that once you have the right answer or this amazing insight, you're almost at the finish line. You're almost able to drive whatever improvement or gain from the insight. I see a lot of internet content describing obtaining actionable insights. You want to drive improvement with data, and getting actionable insight is step one of three. To drive improvement, fundamentally, you need to get people to do things differently on the shop floor. Suppose you have this pure, accurate data set that incorporates machine content and people's insights. In that case, the next thing is you need to make sure that people perceive this data to be true. You need to make sure that folks on the shop floor trust it. This element of trust is so critical and so important and often overlooked by technology providers because if folks don't trust these digital systems, they're not going to act on the shop floor. To act, you first need to trust that the data is true; you need to change their perception.
Manufacturers often have a long history of incomplete, inaccurate, and untrustworthy data, which I saw when I was in manufacturing. You show an operator a report and see their eyes roll. In your data, you don't see people's eyes rolling; that doesn't pop up. Suppose you can change your perception and ensure that people trust the data. In that case, you can hope to change your behavior to improve performance. The two things here are the data set that often manufacturers and other industries believe is the complete data set often only scratches the surface. The second thing is that most organizations are today and will be fundamentally people businesses for a long time. To drive improvement, you need to find a way to change the perception and drive the behavior of people working on the front lines.
[00:07:36]
Ken: If I were to summarize your step one, it is about providing context for the data. Not just the data itself but meaning. Number two, making that meaning actionable for those empowered to do so, i.e., operators. Three, doing that consistently enough to create trust that the data is solid and that the feedback loop in their operations is known and trusted in that as well. All of that converged on to Raven AI, which you founded in 2015. You referred to Raven's value proposition as automated contextualization and OEE software for manufacturing- manufacture, excuse me. For the laypeople among us, can you describe OEE and then discuss what automated contextualization is in practice?
[00:08:26]
Martin: Yes, so for OEE- and we don't need to get into the nuts and bolts of OEE, but fundamentally, it's a measure of how efficiently you're using your equipment. How often are you producing when you are scheduled to produce on a particular machine or process? The things contributing to OEE are time lost because the machine's broken, you're waiting for resources, and you're spending too much time to set up. It is an efficiency metric understood by manufacturers. I would say it's not far off the QB metric in football at the NFL, where people know that is something you should focus on. But if you get them to show you exactly how it's calculated, you may get some differences of opinion. But fundamentally, it's a metric that captures the performance of a particular manufacturing process. Now, to calculate OEE- and there are a bunch of other metrics that manufacturers use to measure their performance, you need to be able to categorize time.
You need to understand how much time was spent producing, how much time was spent setting up, and how much time was lost. The starting point for that metric, and many other metrics, is a timeline. Now, to create a timeline- you can think of a timeline as a general ledger. If you're looking at financial data, your general ledger shows revenue coming in and expenses going out. Before drawing insights from that kind of data, you need to ensure that you have fully captured all the ins and outs, and that's the starting point for Raven. We want to understand how every second of production's time was spent. We can get some of this information from machines, but when the machines aren't running, you often need to get feedback and insights from operators. You can imagine that 300 segments of time need to be accounted for in a day. One of the challenges with systems that do this- manufacturers have been trying to account for time for a long time, and many of these segments need to be categorized by people on the front lines. One of the things that people on the front have a deep instinct for is that their primary job isn't to account for downtime. Their primary job is to do their job, which is to help produce goods for the customers. What happens is that many of these downtime segments needed to fill out this full accounting of what happened in the day are left unfilled because operators don't have the capacity or desire to distract themselves from their job to categorize that. Automated contextualization is effectively the ability to categorize or contextualize that time segment without asking the operator questions.
I always remember returning to my time on the shop floor of a manufacturing plant; we can get into this later. But when we were developing Raven, I always thought, when you need to ask an operator a question with software, how would that question be perceived if you were standing next to the operator asking that question? Every five minutes, "Are you still in the setup? Are you still in the setup?" The idea here is that we automatically contextualize a significant portion of these downtime segments to minimize the burden on the operator. The ultimate result is being able to categorize most of the time, which is very difficult in our industry. This is one issue many companies struggle with; it's not complicated to calculate OEE. It's complicated to develop a complete and accurate data set that feeds OEE metrics and other systems like ERP and MES that rely on complete and accurate data to plan and operate the business and understand performance.
[00:11:52]
Ken: Okay. I know Rockwell has recently been using this theme of automation-to-augmentation. It was interesting because you talked earlier about augmenting the operator. In some sense, automating contextualization, i.e., not requiring the operator to answer constantly, the system is, in some sense, augmenting them, and so I can see how all of these pieces come together. Perhaps to put a point on this, can you tell us a little about some of your notable use cases and wins?
[00:12:22]
Martin: Maybe to go back to that augmenting, which is a key thing here because, in some ways, many systems view operators as a source of data that needs to be instrumented. Operators sense this. In effect, what I alluded to earlier was the fact that a lot of these insights that can help manufacturers focus on the right things are in the heads of operators and are things that only they see. The augmenting capability, one of the key things we do for our clients and is a key thing that unlocks huge amounts of value, is augmenting the influence of operators at the frontlines. When I say operating- augmenting their influence effectively, I always like to imagine that Raven is a way to give operators this long, pointy stick that if they need to poke a part of their organization to work on something or a problem that they see, it's their way to influence. Whether or not that's influence maintenance to come and fix an issue that they see now, influence engineering to address a problem with the process, or influence the organization to purchase a particular piece of technology, there's an untapped capability that is often categorized as this eighth waste. But the capability of operators on the shop floor, who have access to data you can't get from digital systems, is massive. In the context of Rockwell, it may not be exactly how I've just described it.
Still, there's a huge capacity to be unlocked and augmented by connecting the insights and ingenuity of folks standing in front of the process to the rest of the organization. You asked about use cases for Raven. Once you can unlock these insights from the front line and create that fully contextualized general ledger of time on the shop floor, you begin to see where the biggest opportunities for improvement are. Often what happens- and maybe I'll describe the baseline. The baseline for manufacturers is being able to account for 80% of the time, but that 20% of the time that's not accounted for is, in some cases, half of the downtime, which makes it very difficult to know where to start and focus your efforts if you're trying to reduce downtime. Once our clients see contextualized time- say 98% of the time contextualized, they can see where their true biggest opportunities are. Often, their biggest opportunities are a combination of things happening at the machines and in their process. For example, one of the most common things for our clients to see is their biggest opportunity to drive improvement is related to a waiting loss. Waiting loss could be waiting for maintenance to arrive at a machine, waiting for the operator to come back, waiting for somebody to move apart, or waiting for resources. These waiting losses won't be seen if you only look at PLC data.
For one of our clients, part of Danaher- one of the issues they found was they're losing 1500 hours per month waiting for maintenance to arrive. Once you identify an inefficiency as simple as waiting, organizations leveraging lean manufacturing principles and understanding how to drive improvements will address that relatively quickly. The challenge isn't the capability to drive those improvements; the challenge is to sift through all that data and find that lowest hanging for improvement. Now, for many manufacturers, these operational losses are the first issues they see. There's too much time spent in setup, waiting for resources, and issues with shift change. As you progress and address some of these operational losses, you'll reach the point where the natural next step would be to bring in best-of-breed technologies to address the next loss. Then those kinds of solutions could be to bring in a robot, a vision system, or something to bring your process to the next level. Simply put, the value we provide is helping manufacturers understand exactly what's happening now and what has happened in the past, which is the first step to driving improvement as part of digital transformation. You need to understand the basics before you jump to solving problems.
[00:16:29]
Ken: It seems like such a simple value proposition in some sense, but in practice, especially given the way automation systems have been developed, very complex to bring that out and, more importantly, to provide that contextualization in there. Let me ask, how do you know when an organization is ready to adopt your solution? What are some of the best practices you've seen in realizing that potential value?
[00:16:53]
Martin: I think many organizations are way more ready to transform digitally than they believe. In some ways, this is because the kinds of conversations about Industry 4.0 that are most exciting are things that many manufacturers aren't ready for. For example, suppose we were to have a podcast about predictive maintenance and predictive performance. In that case, everybody wants to be able to predict the future and know what actions to take right now to avoid a future poor performance, which is the bleeding edge of technology. Now, to get to the point where you're able to predict what's going to happen, you need to start at the basics and the basics regarding driving improvement with data. The first one is, do you know what's happening now? Do you know what's happened in the past? Do you have that complete and accurate data set to describe that? The next one is once you have that accurate data, you need to analyze that data to uncover the reasons why performance was good or bad. Then, can you begin to take action to address future performance? Now, if you were to go to the shop floor of any manufacturing plant and chat with their supervisors, the plant manager, and operators, and talk to them about the value of being able to understand what's happening now and has happened in the past. That's something that many people can wrap their heads around.
You don't need to begin to talk about the next steps. How will we use these two years after we've addressed our inefficiencies? By starting things in a way that's understandable to the shop floor and understandable to operations, it's much easier to create engagement. Once you have engagements and are committed to driving improvement with technology, those are the two ingredients you need to drive improvement with Industry 4.0. I think, in some ways, a lot of the narrative around the barriers to Industry 4.0 adoption is around technological capabilities: they're lacking in manufacturing plants. In some ways, I think that gives a pass to technology providers that have made technology too difficult for manufacturers to adopt. Manufacturers know how to drive improvements if pointed in the right direction. As with any initiative, you need to start with the basics. One of the basic things for anything that requires people to change is your focus on change, engagement, and getting folks using this onboard. For us manufacturers that have already demonstrated the ability to drive gains with lean manufacturing principles, who have an engaged shop floor interested in solving problems, that is the barrier, and that's what we look for in our clients. Technological capability- that's something that should be the responsibility of technology providers to make sure that their solution is, from a user perspective, simple enough for folks in manufacturing to understand. There's extremely high value in things that are useful to the frontlines. People will use it and get value from it if it's useful.
[00:19:47]
Ken: We've discussed manufacturing in the initial use case. What you guys have done was certainly enough for us to warrant an investment because we know firsthand the needs around factory floors, especially in this Industry 4.0. Even as we move to Industry 5.0, at least the way the EU sees it, people and the planet are now woven into the overall productivity thesis. I think what we were pleased to see in a lot of the due diligence we did is that you seem to be having an even greater impact on the supply chain, particularly, as I remember, warehouse operations. Can you tell us a little bit about this use case?
[00:20:31]
Martin: Yeah. We're supporting a very large, well-known organization with their warehouse or shipping operations. The application is particularly looking at what's happening at the loading dock. Trucks arrive in the loading dock; the door opens, and people unload and load trucks, or in this case, unload trucks. It's a challenge today to get folks to work in loading docks and to do that efficiently. What we're doing with this application is helping them understand what is happening at the loading dock door. Are they unloading a truck? Are they waiting for somebody to bring them paperwork, so they can begin to unload the truck? Are they waiting because they don't have the forklift? What is that story of the day if you were to stand next to the operator all day? This is one of the things that I recognized when I worked in manufacturing. If you had a plant manager standing or an engineer standing next to each operator, what would that engineer see, and what would the operator ask them to do? You can imagine how efficient that operator would be if their engineer looked at the process in that way. In this warehouse application, we're helping them to understand how time is spent. The ultimate result is that they understand where they are inefficient and can now produce or process more trucks with the same number of resources because they understand their inefficiencies. This highlights many different types of processes in and outside of manufacturing benefit from understanding how time is spent. If you truly understand when time is spent productively when time is lost, it is relatively straightforward to address the issues once you understand. You need to define the problem.
Once a problem is defined- there's a quote, no. It's almost more than half-solved. In this application, manufacturing is our core; we understand it, the supply chain- huge opportunities. During the pandemic, we supported some hospitals in Nova Scotia with an application to watch how time was spent in an OR. This contextual timeline, or this general ledger for time, is applicable in manufacturing. Still, it's applicable in many industries. The challenge for many organizations is constructing this contextual timeline with hard data from digital systems and mushy data from people and then presenting it back to those people in a way they trust and understand.
[00:22:52]
Ken: Yeah, I fully agree. Thus, I think was the real long-term interest in you guys because I think this area, call it 'process optimization,' in some sense, has applicability to quite a few domains. I was also happy to hear about the hospital one because I've seen several attempts at effectively applying lean principles to hospital operations. Let me ask, where do you see the greatest opportunity areas for this- I'll call it 'process optimization' in the next five years?
[00:23:23]
Martin: Well, it's exciting in manufacturing to drive improvements on the shop floor. But in some ways, if you look at manufacturing organizations as a system, optimizing one area of the system does not necessarily optimize the system. What's exciting to me is that as these different areas of organizations adopt best-of-breed technologies to improve performance, some of these systems connect. Then, rather than isolate optimizing in corners of the organization, you optimize one thing, and really, what needs to get optimized for manufacturing organizations is you need to optimize that flow from the operator helping to produce the good at the machine to the consumer making the transaction at the store or on the internet. I think I've always said that it'd be great the day that the kind of software that we're talking about today, Ken, is an app on the Shopify store for Shopify vendors to optimize their operations. We have all the data; it needs to be connected so that one data set captures an organization. Once you have that, there are huge opportunities to drive efficiencies. Suppose you're able to drive operational efficiencies as I've described. In that case, one of the effects is that you can likely move away from having massive manufacturing facilities in a few locations worldwide. Now, suppose you can do things in a much more efficient fashion. In that case, you can have more localized manufacturing because you can do things more nimbly and efficiently. This also links to some of the stuff we do with our Industry 4.0 Club. We're excited about how Industry 4.0 has the potential to improve profits for manufacturing, improve the experience of frontline workers, and improve the experience of consumers. Making things more efficient also makes things more sustainable. In some ways, there's a win, win, win scenario here if we can get the organizations to adopt Industry 4.0 in the way that it can be if people look at it in a holistic way rather than as a tool to provide localized efficiency gains.
[00:25:29]
Ken: Back in my control systems engineering days, one of the earliest books I read on the manufacturing process was the famous "The Goal" by El Goldratt. As you may remember, it was called a 'theory of constraints at the time. But there were some other names that I thought were interesting. 'Congestion theory,' 'throughput management.' It all had to do with effectively manufacturing shop operations, and about the time you open a limit in the flow on some machine tool, the rest of the processes, the limit's just going to change to another part of the process because it's all a flow effectively. In essence, it's interesting. We call it Industry 4.0 or 5.0; there are many topics, but the general theories have been well-established for decades if you look back. I think it was 1979 when the first part of that was written. You mentioned a moment ago with the Industry 4.0 Club, and I'm glad you brought that up because I'm curious. When you're not running Raven AI- because we're an investor, we know you should be doing 200% of your time. But during that 1% that's left, I know you're running the Industry 4.0 Club. The mission is 'Accelerate the evolution of Industry 4.0, delivering better experiences to consumers, better profits to manufacturers, and better jobs for factory workers.' Tell us a bit about this passion and community you've developed.
[00:26:48]
Martin: Yeah. I want to start by saying that I'm a co-founder of that club, and a lot of stuff happens based on the efforts of others driving that. But yes, the club came about around when I met you when we were all stuck at home because of COVID, and I was reaching out to begin connecting with people on LinkedIn first. We started connecting on Clubhouse, and effectively, there's a huge community of folks who are interested in Industry 4.0, and in some ways, because the pandemic made it so much easier for us to connect digitally, and we leveraged some of the things that were taking off at the time here. We're able to create this global club of folks interested in talking about Industry 4.0 in a way that could help move things forward. I remember being in a Clubhouse room with myself, and then I picked somebody on LinkedIn to join me. Then within a month or two, we had rooms with up to 5000 people listening in, collaborating with Hannover, Messe and IoT World, and other organizations. It grew very quickly, and now we have over 20 co-founders. We collaborate with events around North America and Europe as well. It's exciting to have a group focused on driving meaningful change.
The group itself has representation from the manufacturing plants. We have somebody who's a supervisor at a medical device company. We have representatives from tech. To drive meaningful change, it must be with groups representing different manufacturing players, which our group does. Yeah, it's been exciting to get to know the other co-founders. It's been exciting to see the reaction and the interest from folks around the world in Industry 4.0, but really, in the kinds of changes that Industry 4.0 can bring. A lot of people have an appetite for doing things differently. I was at a conference a couple of days ago with one of our clients, describing that when he hit the shop floor, he was shocked to see things being done in a way that he's pretty sure was the same as when his grandfather was there. There is a better way, and for us to break through and change the perception of manufacturers of what they can achieve with this kind of technology, this problem needs to be addressed in multiple ways. Industry 4.0 Club is about talking about the potential and our excitement in some of the challenges to get manufacturers to adopt Industry 4.0 in a way that will benefit them. It's been a great experience. I spend as much time as possible there, but as you mentioned, I am quite busy with Raven. Suppose anybody listening wants to check it out Industry 4.0 Club; we have events on LinkedIn- I'm not sure if we're on Clubhouse anymore, but we've also started to participate in events live.
[00:29:27]
Ken: Excellent. While these seem like different activities, they're quite complimentary, and both speak to your role as a connector. I mean that in the sense that all stakeholders, not just executive ones but frontline workers, the mid-tier managers, everybody that has a stakeholder potential in the problem set you are including reaching out to. It's interesting to see how the software in the club has a close alignment on that. In closing, I always ask about inspiration, books, etc. How do you find your day-to-day inspiration?
[00:30:05]
Martin: I love seeing when people can perform at high levels, whether it's sports or business. It's exciting to see people who have figured out how to perform at a high level. To do that- it's one of the things that is exciting with technology. To perform at a higher level, folks, in some cases- it doesn't have to do with your natural capability; it has to do with your understanding of the process and access to information. The example I always love to bring up is Alex Honnold, the climber there who free-soloed. I don't know if you've seen it. If you think about what that guy could do, he could climb a massive rock face without any gear. But there's nothing different between him and somebody who was a climber 200 years ago.
What's different is access to information. While he was training, he got all the insights from climbers around the world. The idea that with technology, he could perform at a much higher level- this is the same thing that excites me and motivates me when thinking about myself and my day job. Using technology makes it easier for people to perform at a higher level. Effectively, the use of technology to commoditize excellence. I love hearing stories of people performing at a high level; when they describe it, it's not magic. It's a process. It's exciting, and I think that drives me personally and in my business. What can we do- for me to perform at a higher level and enable others to perform at a higher level and use technology to achieve that?
[00:31:35]
Ken: I love it. "Commoditizing excellence." I think I found the subtitle for our podcast. That's a great one. Martin, thank you for sharing this time and these insights with us today.
[00:31:45]
Martin: Thanks for having me, Ken.
[00:31:46]
Ken: Absolutely, it's been a real pleasure and a great conversation. This has been Martin Cloake, CEO of Raven AI, commoditizing OEE excellence in manufacturing and supply chain. Thank you for listening, and please join us for the next episode of our Digital Thread podcast series. Thank you and have a great day. You've been listening to the Momenta Digital Thread podcast series. We hope you've enjoyed the discussion, and as always, we welcome your comments and suggestions. Please check our website at momenta.one for archived versions of podcasts, as well as resources to help with your digital industry journey. Thank you for listening.
[The End]
Connect With Martin Cloake
What inspires me?
I enjoy seeing people perform to their full potential, whether in business or sports. I am inspired by high achievers. In some cases, your performance is determined by your understanding of the process and access to information rather than your intrinsic ability. That's what's so exciting about technology. A famous example is Alex Honnold, who free-soloed. He could scale a big rock without using any equipment. There is no difference between him and a climber from 200 years ago.
What is different is the availability of information. While training, Alex Honnold learned everything he could from climbers worldwide. The idea that he could perform at a much higher level with technology excites me when I think about myself and my day job. Using technology allows people to perform at a higher level. In other words, the use of technology to commoditize excellence.
About Raven.ai
Raven is a manufacturing technology leader that helps companies empower the frontline and improve operational performance with our automated contextualization and OEE software. Raven combines and analyzes data from machines and operator input to provide a real-time, meaningful timeline of events with context for everything happening on the line — accounting for 100% of production time and OEE losses. By replacing manual pen-and-paper tasks with smart devices, operators can tag the exact reason for downtime and inefficiencies with the touch of a button. With round-the-clock data collection, Raven's visual UI displays real-time alerts to drive what actions and improvements to take next, driving continuous improvements across plants. Powered by AI-based cloud analytics and IoT/edge devices. To learn how leading manufacturers like Walmart, Danaher, Michelin, and Coca-Cola are accelerating continuous improvement and enhancing the day-to-day work of frontline teams with Raven, visit raven.ai.
Find out more at Raven.ai.