Jonathan Weiss
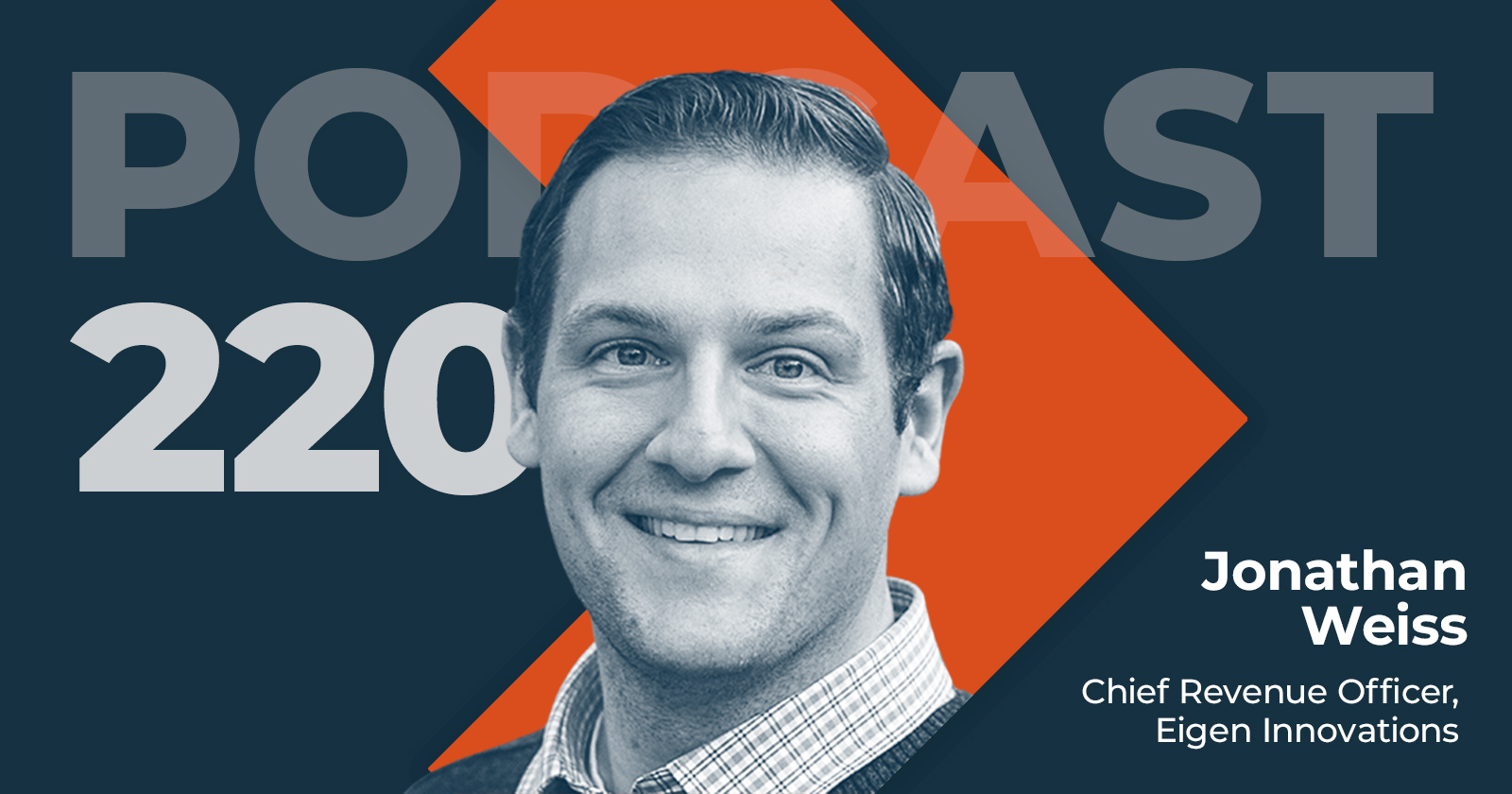
TRANSCRIPT
Ken: Good day, and welcome to episode 220 of our Momenta Digital Thread podcast series. Today, I'm pleased to host Jonathan Weiss, Chief Revenue Officer at Eigen Innovations, who provides industrial machine vision without limits. Eigen Innovations is one of Momenta's early venture investments. Jonathan is a trusted adviser to global industrial clients and early-stage software companies with a proven track record of success in developing and implementing innovative business and sales strategies. He partners with leading manufacturers to drive cost reduction, improve operational efficiencies, and enhance supply chain resilience by implementing advanced technologies like IoT, IIoT, AI, and ML. Jonathan has a strong track record of success, having spent the past decade working with some of the world's most prominent companies, including Amazon, Procter & Gamble, General Electric, Pfizer, PepsiCo, Intel, Koch Industries, and Foxconn, to name a few. He's focused primarily on executing digital transformations in manufacturing facilities, supply chain initiatives, and enterprise applications. Jonathan, welcome to our Digital Thread podcast.
[00:01:52]
Jonathan: Thanks for having me, Ken. It's great to be here.
[00:01:54]
Ken: We were talking a few minutes before, and Eigen was, I believe, our second or third investment way back. I remember seeing Scott, your founder, at an early conference. I believe it was one of the first IoT conferences in Palo Alto. It was Informa, if I remember right, and just being so fascinated with his story, we felt we had to invest early on. You represent the second or maybe even third generation of leadership there, but we're excited you joined the company and are quite excited to have you on this podcast. We call this the Digital Thread podcast, and what we think about is one's digital thread. How did you get to where you're at now? What would you consider to be your digital thread?
[00:02:40]
Jonathan: It's a good question. The digital thread means a lot of things to different people. I guess, in my journey, it's been this conscious effort to create this thread of mine that's all about solving complex problems. I've had quite the evolution throughout my career. I'm sure we'll talk a little bit about that, Ken, but that translates well to Eigen, and it's funny that you phrased it that way where you saw our founder Scott speaking, and you felt compelled to get a seat at the table. It's funny you phrase it that way because I had the same experience when I first came across Eigen. It was through a joint effort I was working on with Intel, and Eigen was brought to the table as a partner by the end of the very first presentation. I knew I had to get involved with these folks somehow, and fast forward about a year and a half, almost two years, and that turned into this full-time role here. We were both impressed with what we saw.
[00:03:39]
Ken: It's interesting to see him in action and understand the impact of vision on the manufacturing space. You guys were very early user of pattern-based vision recognition systems, so we'll get into that in a few minutes. We're very excited to have you. I was intrigued by your early experience—a mix of first responders—so thank you so much for your service and web development. It's an exciting mix, ultimately, that led you to GE Digital in 2015. What ultimately attracted you to industrial automation in general and GE in particular?
[00:04:18]
Jonathan: I grew up with an engineering-focused mindset, so as a kid, I enjoyed watching "How it's Made" and all those kinds of shows on Discovery Channel and History Channel. I grew up in a household focused on education, so I go to museums and things like that. I was always intrigued by understanding how things worked. At an early age, for example, I used to enjoy building computers and stuff like that, and so that naturally evolved and ultimately translated into an interest in the industrial space. What better place to learn about manufacturing than a company like General Electric? Especially since I joined back in 2015, it's been almost ten years. But it was at a time that was very exciting for General Electric; they were starting to think about forming this new digital entity, which, of course, ultimately became GE Digital. They gave birth to the whole Predix platform. I joined at an exciting time; I got to be a part of all of that and see it all come to life, and that propelled my interest and career in the industrial software space.
[00:05:25]
Ken: You were quite lucky; GE Digital is one of those companies I consider to be one of the launching points, if you will, of the industrial IoT. I was relaying earlier that I was a part of Wonderware in the 1990s, and you could say akin to what Wonderware did for HMI; I think GE Digital did for overall industrial IoT. I still love the original campaign, which had the power of 1% and 2%. It describes the efficiency and benefits of applying digital technologies to typical hardcore industries, and it is a great place to call your alma mater. I know you went on to join Software AG, ultimately serving as Vice President for Emerging Technologies in 2019. Can you tell us a little about your role at Software AG and some notable wins during that time?
[00:06:19]
Jonathan: Yeah, sure, I'll explain how I got there. Early in my career, I spent the first ten years as a 'hands on the keyboard' software engineer and then a solution architect, so very, very technical. GE was my foray into more management, people leadership, and focusing more strategically. During my time at GE, I was managing one of the global professional services teams implementing smart factory solutions, and so that translated well to what Software AG was looking for, which was a new leader to come and ultimately run the pre-sales group for the IoT business in North and South America. Pre-sales is essentially the technical arm of the sales group, so think about the engineers, software engineers, and solution architects that support early-stage pursuits and sales cycles. That's the group I was tasked with running, and that quickly evolved into a much larger role at Software AG—I guess, like most things in my career, timing and luck It just worked out that they needed somebody to fill a larger role, and I had proven myself fairly early on in my career at Software AG, so they were kind enough to consider me for that role. Ultimately, I ended up taking over sales and pre-sales. I had full responsibility for everything on the business side of the house for this IoT and analytics P&L. Some of the key wins and some of the things that we focused on there—we spent a lot of time delivering IoT solutions and platforms in the medical device world as well as in the industrial space. We provided platforms like remote asset management and remote and connected field services applications for industrial air compressor companies and industrial rental equipment companies. Then, in the medical device world, probably some of the largest medical device manufacturers on the planet, we were able to strike deals with them and have some impactful successful IoT launches around things like pacemaker configuration devices and stations, smart nurse call stations within hospitals, smart hospital beds, things like that, so it was quite a range of applications that we delivered.
[00:08:33]
Ken: In what I guess I could describe as an archetypical pattern for many industrial leaders, you went from GE to a large technology provider, in the case of Software AG, you can call it an IT provider, and then went on to work at one of the g.o.a.t. hyperscalers, in your case, AWS, as the global go-to-market leader in industrial manufacturing for AWS Strategic Industries. What key lessons did you learn during that time relative to operating at the intersection of IT and OT?
[00:09:06]
Jonathan: Personally, it was an important transition for me. During my time at Software AG, I realized that as much as I love technology and IoT was a lot of fun, I missed being able to focus exclusively on solving problems for manufacturers. It was an interesting hiatus going from GE to more of a general broad-brush approach to solution delivery, or problem-solving, if you will, for various industries. However, the role at AWS appealed to me because it gave me a forum to go back and focus exclusively on industrial manufacturers. So that's why I ultimately left Software AG. It's a great company with great people, but I was fulfilling a passion to return to manufacturing full-time, and this AWS role provided that for me. Some of the lessons I learned early on were: I was reminded just how foundational problems in the world of IoT and data collection are still a problem for some of the world's largest industrial manufacturers, meaning doing what we would consider in technology to be a "basic task," something like collecting data from a machine or a piece of equipment. Still, today, that remains a large challenge for folks in the industrial space. For various reasons, equipment is very difficult technically to connect to due to proprietary protocols; the different types of data coming off the machines and even off of processes are vast and vary. It's all different form factors; something needs to be normalized and standardized, and managing it all at scale across lines and factories is incredibly cumbersome for these manufacturers. It was a good reminder that before we start talking about the "sexy stuff" in technology today—artificial intelligence, machine learning, generative AI, and all these things—manufacturers still need help just getting a foundation developed. That was something I was reminded of very early on.
[00:11:10]
Ken: Certainly apropos. Interestingly, the interest in AI, particularly in generative AI tools like ChatGPT, has created a groundswell of activity over the last nine months for data collection contextualization systems, particularly for industrial, so you'll see we made investments in- we are already in HighByte, but also Aperio around the same lines. I think there are a lot of people realizing that I would love to have those types of- I'll call it user experiences like ChatGPT for my factory process, but the data I have, the control systems I have are just not up to par to be able to be easily mapped to those. You see a lot of companies talking about things like co-pilots as an example and such. It's just an interesting ketchup pattern, if you will, almost whiplash-like.
[00:12:01]
Jonathan: That's right.
[00:12:03]
Ken: Before we jump into Eigen, I wanted to ask- I know most of the hyperscalers we've been watching. Microsoft, AWS, and Google have since retreated from their focus on industrial automation with some well-documented layoffs earlier this year. I'm just curious: why do you think that is?
[00:12:27]
Jonathan: Holy smokes, that's a pretty loaded question, Ken. Each of those companies has approached that a bit differently. AWS, at least on the Amazon front, really didn't see the same pullback that somebody like Microsoft, for example, did. Microsoft went through a round of layoffs where they let go of almost everyone who did what I did at Amazon, so they are your industry experts or subject matter experts. Amazon didn't do the same thing. AWS has some very strategic focus happening at the moment in the industrial sector, so they remain pretty maniacal on growing that business. There isn't a pullback there. I think some organizations get into the industrial space, just generally speaking, and underestimate just how complex and complicated problems are to solve. It's very similar to regulated industries, where you have a lot of sensitivity around the types of data that can be extracted from machines or processes, a lot of proprietary datasets that manufacturers are uncomfortable sharing on Cloud platforms, privacy concerns, and security concerns. By the way, Ken, we can debate the legitimacy of those concerns; that's probably a topic for another day. But these are some things that are top of mind for manufacturers. Companies come in with an expectation that it will be quite easy to tackle some of these challenges, but in reality, it's not. It's complex stuff. Also, it requires much more than just technological expertise. It requires domain expertise, like trying to sell technology or provide technology for any very specialized niche application, going into a process manufacturer versus a discrete manufacturer, pharma versus CPG, food and beverage, metals, and so on. You have to talk to these folks differently, and you have to understand their needs quite clearly. The needs differ from manufacturing function to function, if that makes sense. Without that domain expertise, which, quite frankly, a lot of those companies didn't have, it isn't easy to get buy-in from the executives at those industrial companies. Hopefully, that makes sense.
[00:14:52]
Ken: Yeah, it does. You've hit on some great points in there. Traditionally, when considering the "IT-OT" divide from a technology perspective, you should be able to bridge it with technologies, right? Plenty of solid IT technologies will do what OT needs them to do. But what they underestimate, and you hit on it with the word sensitivity and domain expertise, is the conservativeness of the culture there. Jokingly, nobody ever got fired, putting Siemens or Rockwell PLC in their plant. It's just how things have always been done. Especially, as you say, in highly regulated industries where the cost of maybe an hour of downtime outweighs any savings you could potentially get from "moving from proprietary hardware and software systems to more open ones." It's that sensitivity, the culture if you will, that is the part that I see an awful lot of people underestimate; thus, I appreciate people who early on spent their time on factory floors because they also appreciate when the alarm goes off to say that the process has stopped the fear factor that goes in with that. All of this, of course, is trying to paint a broad picture of the experience that you're bringing into Eigen. I was so excited a couple of months ago when I saw that you were named Chief Revenue Officer there. As mentioned, we're an early investor in the company. Tell us a bit about the company and what attracted you to it beyond, as you said, seeing Scott in action.
[00:16:21]
Jonathan: Yeah, absolutely. A little bit about Eigen- historically, just for some context. Eigen was born in academia, and you see that today just based on the sheer talent that remains in-house. We have quite a few PhDs on staff. Folks are incredibly smart, and that's one thing that intrigued me about the company very early on. As I mentioned, Eigen started out in academia. It was a research project at the University of New Brunswick that ultimately became its own entity or its own company, and that was 10 or 11 years ago now. The company has been going at this for over a decade, and there's been an incredible amount of innovation that's taken place over the last 10 years, some phenomenal proprietary technologies and patented technology that the company has created. Quite a bit of that was what intrigued me, just how much 'secret sauce,' for lack of a better term, existed here and the power that it delivers for Eigen's customers. The ability to do incredibly fast image recognition and quality inspection within factories and the scale at which we do it. Some companies are using Eigen technologies to inspect quite literally millions upon millions of parts every year in their facilities. Some of the highest-volume folks using our stuff are doing 30,000 or 40,000 parts a week that get inspected. You start thinking about the scale of this solution and what I can deliver- that was incredibly compelling to me because, in my experience, getting started in this space doing anything in industrial IoT is not typically the problem. Where you run into issues is breaking out of your pilots or proof of concepts and implementing technology at scale; that's where many problems reside. Seeing Eigen was able to mitigate that was quite compelling to me.
[00:18:19]
Ken: You describe Eigen Innovation as "Industrial machine vision without limits," a phrase that I absolutely love. What does that mean in practice?
[00:18:28]
Jonathan: It quite literally means what it sounds like. We've created a toolset that empowers manufacturers, system integrators, and vision teams. For anybody who cares about leveraging machine vision, we empower them to do anything that they can think of. We built this tool specifically to be hardware agnostic, so you can leverage just about any camera or Edge hardware that you'd like, and you can address just about any use case with a tool. We have the exact same software tool running to do things like inline inspection for injection molding processes, and then you'll find the exact same tool looking at, for example, high-gloss class A style automotive surfaces for bumpers and things like that, or even inspecting metal welding processes using thermal cameras. It's quite versatile. Hopefully, that makes sense.
[00:19:22]
Ken: It does. I remember the company's roots were very much regarding infrared thermal cameras. One of the first use cases I saw was a quality control one testing the rear window defoggers or defrosters of automobiles coming off assembly lines, so basically, as heating the windshield and across as you would expect it to do. I also remember some interesting ones with large paper manufacturers looking for early tears in manufacturing paper. Very interesting stuff, but I'm curious. What have been some of your more notable use cases and wins?
[00:20:00]
Jonathan: The paper one's a great example because it's quite quantifiable, and my gosh, you've got a great memory, Ken, because this was definitely from a little while ago. But they still use our solution today in production, of course, and that's a great example because it speaks to a few things. It speaks to the value that technology like this brings to manufacturers, but it also speaks to the speed at which we can do what we do. This company saves about a million dollars a year, more than a million dollars a year, due to unplanned outages that they can now mitigate and equipment failure. They're able to save that money because they used to run anywhere from, say, 7 to 10 plus outages per year per line related to excessive build-up of coating on that paper. It's a very high-grade specialty paper they use, and what happens in the process is a continuous process. A very large spool of paper, it's constantly going down a line, if you can picture that. As it goes down, this specialty high-gloss coating is applied to it. However, a failure mode can occur where that coating is not applied evenly and is at the millimeter or submillimeter level. But if it's not applied evenly, and there's a build-up that occurs on the equipment itself, it only takes about eight seconds- in some instances, 10 seconds, but it's quite quick, that will actually damage the equipment and cause about $100,000 worth of damage every single time, and you only have about 8 to 10 seconds to catch it. When you think about the speed at which this has to happen and why technology like this is essential, well, for one, that coating- the human eye can’t see that kind of uneven build-up. That's why we leverage technology like ours. Especially in this case, we use thermal cameras to give us the appropriate view we need. But yeah, tremendous cost savings. The defogger is a good example- using camera systems, we could completely augment the manual process of applying a heat-sensitive paper that they would put over those rear windshields. Traditionally, you apply the paper, put an electrical current to the window, and then see those defogger strips turn on. Then, you look for demarcation on that paper to understand whether you have good or bad strips. We removed all that by using one thermal camera, and now that process takes seconds versus minutes. We've got many great use cases and applications in the world today like this.
[00:22:33]
Ken: You mentioned earlier the quintessential challenge with many solutions in the OT space, and that is how to go from proof of concept to pilot to add scale. You guys clearly have cracked that nut, and so I'm curious: how do you know when an organization is ready to adopt your solution? What best practices have you seen in them realizing that potential value?
[00:22:57]
Jonathan: the answer I will give you applies to much more than just what we do. It's more of a digital transformation answer but very relevant. It all starts with a clear understanding of an organization has need and the quantifiable impact of resolving that need. Some folks call that value engineering. It has many different names, but it's fundamentally all about understanding the opportunity cost, and if we don't solve this problem, what does it cost your business? If we solve this problem, what's the benefit of having that quantifiable material impact value proposition for your business? That's incredibly important. Quite frankly, without having that, what happens is you get stuck in this rut of doing POCs and pilots on what's likely going to be an unfunded initiative that does not have the right level of executive sponsorship because there's no business case or justification around the value of the investment. It all starts with that; it starts with a clear understanding of the need and the value of addressing that need. If you can do that right, I would venture to say just about any project will be successful from an adoption perspective to get to scale. In our world, the critical difference for machine learning in vision systems and cameras is that we've cracked the nut on empowering our customers to build a machine learning model once and then deploy it just about anywhere they want to do similar inspection methods. Customers who make a widget train the machine learning model to inspect it. Then, they want to inspect the same widget on a different line or in a different facility. We make it a super simple process to point and click to deploy those models repeatedly, which traditionally is a very large barrier to scaling these vision systems.
[00:24:51]
Ken: It captures well, in fact, closes the loop that started in GE Digital for you, which was the focus on outcomes. That was probably one of the first times I heard that term describe the 'why' of digital transformation. You've done a good job of weaving that into your current work. In closing, I always like to ask: where do you find your inspiration?
[00:25:16]
Jonathan: In a variety of places. I'm still a nerd at heart, so I still love reading engineering magazines and different vision publications that are more relevant to my role today. I still love reading stuff on LinkedIn; I follow a lot of the big guys like Rick Pallotta and all of these people who are constantly publishing stuff, as well as some of the analyst firms. But I'm pretty big on reading just about everything I can, and to be honest with you, I like challenging the norm and formulating my own opinion. I like to go to a couple of different sources, often conflicting sources, so I like reading the comments on LinkedIn. It's always fun, for example, when somebody like Walker Reynolds publishes something, and you start reading people that challenge him. There are a lot of interesting insights floating around, and I guess, like most things in life, the truth is somewhere in the middle. That's how I look at it. Then, outside of work, I'm a creative guy, so I love music. I love playing drums and guitar in my spare time, which unlocks some creative juices for me as I think about the problems I must solve for my customers. Sometimes, I need to disconnect and come back later. That helps me as well, personally.
[00:26:24]
Ken: That's nice. It's funny talking about extremes. Walker Reynolds and Rick Pallotta. I'll leave it at that; those are the most entertaining, as you say, comment streams coming out of some of the stuff on LinkedIn.
[00:26:37]
Jonathan: There's no shortage of entertainment, that's for sure.
[00:26:39]
Ken: Oh no, no, no. Clearly, not in this space, but given how conservative OT can be at times, it's probably a welcome respite from the norm of productivity, people, and the planet that makes up so much of OT. Well, Jonathan, thank you for sharing this time and these great insights with us today.
[00:26:56]
Jonathan: That was great. Thanks for having me, Ken. I appreciate it.
[00:26:59]
Ken: Yes. Well worthwhile and very timely, given all the great work that Eigen is doing. This has been Jonathan Weiss, Chief Revenue Officer at Eigen Innovation. Thank you for listening, and please join us for the next episode of our Digital Thread podcast series. We wish you a momentous day. You've been listening to the Momenta Digital Thread podcast series. We hope you've enjoyed the discussion, and as always, we welcome your comments and suggestions. Please check our website at momenta.one for archived versions of podcasts, as well as resources to help with your digital industry journey. Thank you for listening.
[The End]
What inspires Jonathan:
Jonathan stays passionate about technology by exploring engineering and vision publications. He loves learning from LinkedIn, follows influential figures, and values diverse opinions to form balanced perspectives. Jonathan thinks critically; knowing the truth is often in the middle. He expresses his creative side through music, playing drums and guitar for a refreshing break that sparks innovative problem-solving. Recognizing the value of disconnection, Jonathan returns with renewed clarity in his personal and professional life. He blends analytical thinking, creativity, and continuous learning in his multifaceted approach to inspiration.
Eigen Innovations is a company that specializes in industrial machine vision technology. The company’s OneView vision software helps manufacturers reduce quality defects and increase revenue by providing real-time insights, autonomous in-line inspection, and AI-powered industrial technology. Get in touch!