Dr. Alessandro Butté
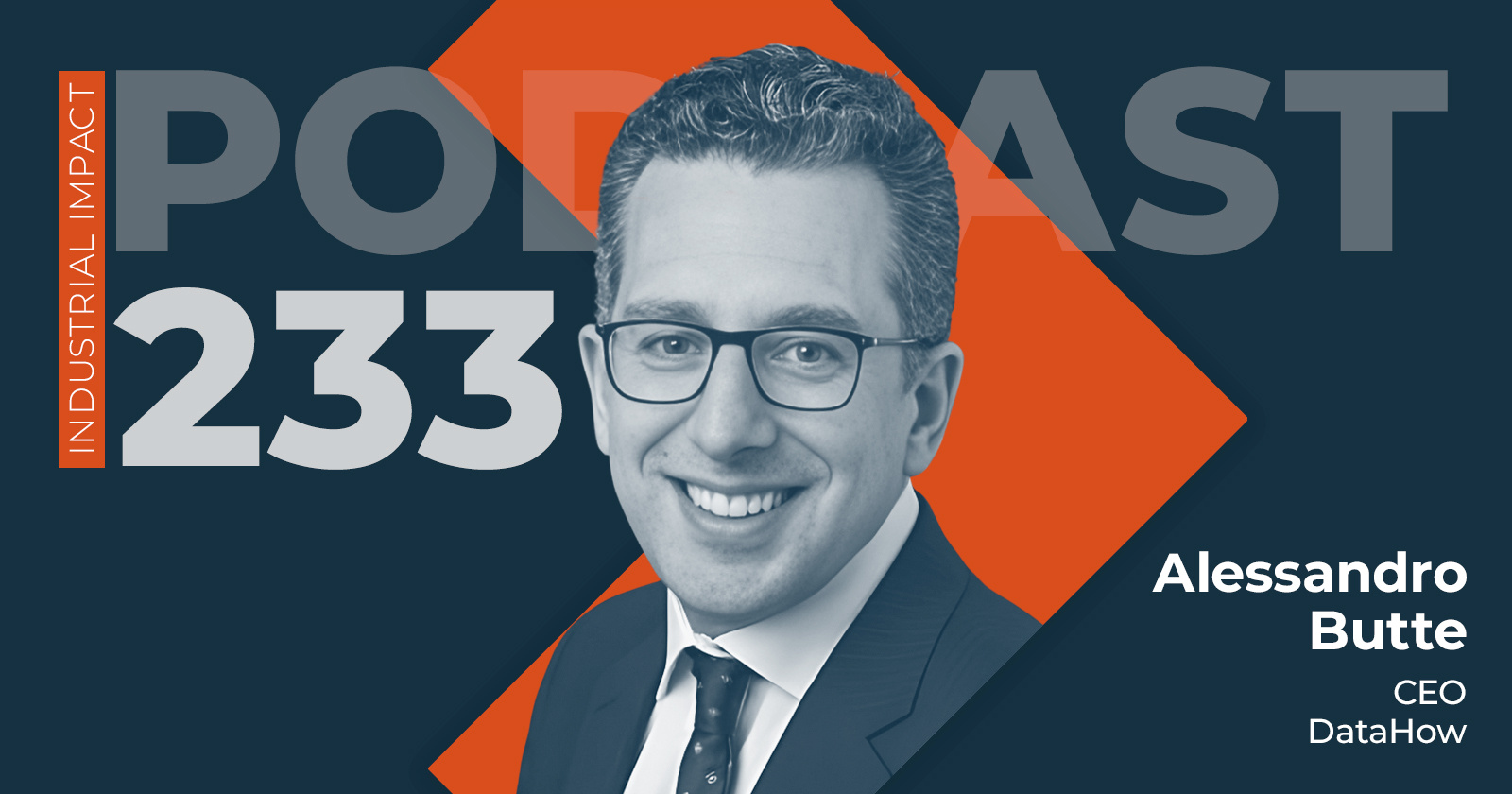
TRANSCRIPT
Ken: Good day, and welcome to episode 233 of our Momenta Industrial Impact Podcast. Today, I'm pleased to host Dr. Alessandro Butté, CEO and co-founder of DataHow, the AI model-based bioprocess development platform. Momenta led the Series A round for DataHow, making it our 54th portfolio company. Alessandro received his MSc at Politecnico di Milano in Italy and his PhD at ETH Zurich in Switzerland, both in chemical engineering. After a postdoc at the Georgia Institute of Technology in the US, he returned to ETH Zurich as a senior scientist and lecturer. In 2008, he joined Lonza as Head of Downstream Technologies in the sectors of small molecules and peptides and was also involved in the pilot program to introduce Quality by Design. He is the author of more than 90 papers in international peer-reviewed journals and several patents. He also completed an executive MBA at the University of St. Gallen in 2015. Alessandro, welcome to our Industrial Impact Podcast.
[00:01:47] Alessandro: Ken, thank you very much for hosting me. I'm very glad to be here.
[00:01:52] Ken: Absolutely. We're proud to have you here and to have closed the investment because, as you can appreciate, it was a long process, mainly because we've brought many great investors into this round. Your technology is so deep and groundbreaking. I think it took longer to truly understand and comprehend it than some of our other investments. Either way, I'm really glad to say we're invested and that you're here and already celebrating the start. We used to call this the Digital Thread podcast and changed it recently to Industrial Impact to better represent what we feel our companies do. But I still like to ask the signature question. That is, what would you consider to be your digital thread? In other words, what are the defining interests and experiences that brought you to this point in life?
[00:02:42] Alessandro: It wasn't a linear path. I went through different experiences, and many times, things developed by chance, by random events, in a sense. As you said, I've always been very passionate about finding solutions to practical industrial problems, especially by combining ideas from different fields to create something new and groundbreaking. When I started Approach Pharma—let's discuss later why—but when I started Approach Pharma, that was the case. My classical chemical engineering background was not enough. At the same time, in the industry, probably still today, but especially in those days, it was very popular. This Lean Six Sigma type of initiative put me in contact with statistics. Even then, I was not particularly satisfied with these tools; we were making too little use of what we knew about the processes but still, very flexible to the problems of pharma. I remember one of these random events where I was discussing with a client how to use statistics, and I showed him that introducing a little bit of chemical engineering knowledge into statistics could dramatically improve the efficiency of these tools. That was the main idea, and then I started developing the simple ideas. Following this path, I met several people, like Michael, my co-founder, or Moritz, who introduced me to machine learning and what we now call hybrid models. From this idea, we started capitalizing, and it was no longer a simple tool to make models for production processes; it became a sophisticated idea to manage data and knowledge in the pharmaceutical industry organically.
[00:04:50] Ken: Your background was tailor-made for DataHow, given how deep your background is in chemical engineering and your extensive manufacturing experience at Lonza, particularly with life science products. What initially attracted you to this bioprocessing space?
[00:05:10] Alessandro: Well, to be very honest, this was not my original idea. I was following in the footsteps of my mentor, Professor Morbidelli, who was a co-founder of DataHow. He is very well known in the chemical industry for exactly what I mentioned before: combining simple ideas from different fields and applying them to completely new areas. In particular, at the beginning of the 2000s, biological production—in pharma and general—was clearly the new frontier. It was also evident that chemical engineers were largely excluded from this field, which was seen as the domain of biotechnologists and biologists. At that time, Professor Morbidelli applied a very simple rule of chemical engineering: if your process has low productivity, move from batch production to continuous production. This approach led to the development of continuous processes like perfusion processes and continuous chromatography. I followed up on that and became particularly interested in the FDA's Quality by Design initiatives, which, in essence, follow another fundamental chemical engineering concept: know your process, understand your parameters, and especially understand how this impacts the quality and efficiency of the process. I began applying this to bioprocesses, which was quite innovative at the time, and this, as you mentioned earlier, caught the attention of Lonza, who then hired me to do that.
[00:06:51] Ken: Our listening audience is quite familiar with traditional discrete and process industry manufacturing. How does bioprocessing differ from these domains?
[00:07:02] Alessandro: The typical idea circulating, especially from the pharmaceutical industry, is that the regulatory framework is the main difference between pharma and any other industry. Frankly, I've always been skeptical about this notion. I find it overly simplistic and unjustified. I've seen other highly regulated industries adopt groundbreaking technologies. However, it is indeed true that in pharma, particularly in biopharma, the way of developing a new drug or product is very different from other industries. We must go through a complex clinical phase requiring significant time and capital. The time we have to develop a manufacturing process is actually very short, with limited resources. This makes the pharmaceutical industry relatively different from other industries, requiring everything to be faster, more flexible, and more difficult to apply complex technologies. In the end, it all goes back to another financial truth: for most industries, the cost of goods is a major factor, but it's not where pharma makes its money. This is another general problem in the pharmaceutical industry. At the same time, there is a huge focus on managing a portfolio of drugs, but traditionally, very little focus on managing or improving manufacturing. Risk management is everything; not failing in production is paramount, and this is the major concern.
[00:08:53] Ken: That's an interesting point, particularly regarding the cost of goods going into the products. I hadn't quite considered it, but the finished goods are worth extraordinarily more than the input products, giving you much more flexibility in getting there. This brings us right up to DataHow, which you co-founded in 2017. What inspired the team to start DataHow?
[00:09:20] Alessandro: In the end, the idea was simple. Again, to mitigate every risk in creating a manufacturing process for a new drug, especially in biopharma, we moved to what we call "platform processes," where processes can be repeated similarly across different drugs and scales. This is a very important concept, and our belief was that the ability to manage knowledge and information across different drugs and scales for the same platform process was not an additional risk that pharma had to engage in by adopting new technology like ours but actually a risk-mitigating factor. The more we manage information across these platform processes, the better, faster, and safer we can produce. We are doing this at DataHow; we develop tools to support scientists and engineers to standardize further the way they develop these processes by incorporating much broader knowledge from data collected across different scales and drugs. It's not just about reducing the number of experiments and saving some R&D money; from our perspective, it's more about empowering scientists to make critical decisions in developing the processes very early with minimal risk, thanks to our sophisticated analytical tools.
[00:11:04] Ken: You describe DataHow as having pioneered the development of hybrid models for bioprocess development, combining hard-coded process knowledge with data-driven AI. In layperson's terms, what does that actually mean?
[00:11:19] Alessandro: Yes, this is indeed very complicated. First of all, for what we are doing, I typically prefer talking about machine learning. Machine learning is an extremely powerful tool capable of finding extremely complex information in the data stream and sophisticated patterns in the data that are not manageable by traditional methods. However, it's no longer trendy to say that machine learning goes with big data. Ten years ago, we talked much more about big data and less about machine learning, but this remains a fact. To learn the features of the process, we need a lot of data, which is exactly what we don't have in pharma. What we do practically by providing mechanistic knowledge, which in the end are just laws of physics, chemistry, mass balances, and all the typical information that chemical engineers use, is to teach the machine learning tool this information so that it can focus on what we don't know about the process—what it has to learn because it's a very peculiar process. This is a nice way of directly seeing it: teaching a machine learning tool. If you want a more mathematical perspective, we are constraining the space of the solutions of the machine learning tool to some physical and chemical properties that must not be violated. By creating these constraints, we dramatically reduce the possible number of solutions and the amount of data needed to create this machine-learning model. In a nutshell, what we are doing with the hybrid models, where we combine mechanistic knowledge and machine learning, is enabling machine learning for small data instead of big data.
[00:13:31] Ken: If one were to measure industries based on their opportunity for digitalization, life sciences certainly would be at the top of that list. You mentioned earlier how that industry differs from others in the sense of regulation, but I'm curious: beyond regulation, what do you think has held back greater adoption of automation and autonomy-based solutions like yours? More importantly, how is DataHow helping your clients accelerate their digital initiatives along those lines?
[00:14:01] Alessandro: Well, to be very frank, I cannot speak for the entire life sciences industry. I can only speak for pharma. The only explanation I can give myself goes back to this financial factor. In the automotive industry, the cost of goods is 80% of the balance sheet. For biopharma, it is sometimes as little as 15%, so the focus on making production processes more effective and more efficient or adopting new technology is minimal because saving 10% in production costs will result in only a 1.5% reduction in the price of the drug. This is the only explanation I can give myself for why pharma is so risk-averse; there is no pressing need to push things to empower competition. What we are enabling our customers to do is be more efficient, especially with regard to risk. How can I be very effective? How can I limit the data, experiments, and information I need to collect but still manage my process very well? My process is very robust. We can create value for our customers by producing a drug that always meets the highest quality standards possible, along with the ability to learn much better about the processes and their characteristics.
[00:15:48] Ken: To help us understand some of your early wins with your customers.
[00:15:54] Alessandro: At the beginning of our journey, we decided to have what is typically called lean product development. It's not about isolating ourselves in a lab or, in our case, an office in front of a computer and developing a product that, in the end, nobody wants or nobody can use. From the beginning, and this was also functional in bootstrapping the company until now, we engaged in many collaborations with the end-users in BioPharma. We have been able to collect a huge amount of data and experience from these collaborations. When we started to disseminate these results very early in our journey, it naturally created a lot of interest in what we were doing. We've also been particularly lucky because digitalization, although varying in emphasis across the pharmaceutical industry, was at the top of the agenda before the COVID pandemic. We were entering a space that was ready to try new solutions. We arrived on time and did a good job.
[00:17:18] Ken: Help me understand, in terms of your clients, how do you know when an organization is ready to adopt your solution? More importantly, what are some of the best practices you've seen in your clients realizing the potential value of your solution?
[00:17:35] Alessandro: That's a very good question, for which, unfortunately, I'm afraid I don't have an equally good answer. One of the problems we see is that even within the same organization, talking with different production sites or business units, the readiness or what we call digital readiness to adopt our tools varies greatly. This is mostly driven by local initiatives and so on. In general, we have seen that adoption is much faster in industries where these initiatives have strong coordination at a very high management level. It's not just about modeling processes; you have to create an entire digital framework from the supply chain to manufacturing, to data collection, creating digital twins as we do, and ensuring coherence across different stages of the drug life cycle. When there are coordinated initiatives, and unfortunately, this is not always the case, the results are better. In particular, when the industry allows scientists to assess the technology fairly, sometimes daily duties and R&D activities or exploring new ideas happen simultaneously. Of course, daily duties always take priority, but exploring new ideas is fundamental. Empowering scientists to challenge the technology and take risky actions is the best way to assess the technology.
[00:19:23] Ken: I firmly believe it when I mentioned groundbreaking in my introduction. It's not simply the technology per se but the approach to utilizing that technology, the hybrid modeling in a domain that is probably not as comfortable with some of the current digital innovations. As we said, for several reasons, including the financial factor and, of course, the regulatory aspect. But those wins are changing, and you seem well-positioned to scale up. Part of the problem is always finding the right consistent buyer influencer in an organization, especially where you have to raise the tide of knowledge. So, you are particularly playing a pioneering role in your company as well. It's a worthwhile bet on this groundbreaking side of it, so we look forward to working with you on that. The industry is at an important crossroads when we talk about reshoring, the energy transition, labor challenges, and what's happening in the defense sector, especially in Europe. What are some of the key trends you're watching relative to the life sciences space?
[00:20:43] Alessandro: Well, this is, again, a very good question, although rather complicated. But I can take part of your question. For sure, reshoring and energy—most of the time, they come in the form of sustainability—are two major topics, especially in pharma. We have seen during the recent pandemic how vulnerable our supply chain can be after many years of optimizing the global scale of the supply chain. But also, the recent war in Ukraine is a challenge in another direction, for instance, energy. A lot of work is done there. Still, again, I firmly believe that all of the efforts that DataHow is putting in place to move or enable the industry to move more effectively and rapidly to what we call Pharma 4.0 will be an important part of the overall solution. By enabling all these digital possibilities through more effective data management, we can handle the quality of drugs much better at any stage of the drug life cycle. We can be more rigorous in defining and creating better ways of producing high-quality drugs while also being more efficient because of these tools. The moment that we know better, it will be simpler and more effective to scale out small and local productions. This will significantly shorten the supply.
[00:23:04] Ken: Perhaps at the risk of throwing a bit of a curveball, you are one of our Swiss-based investments, particularly sitting in Zurich. I know you're very close to the university there. I'm curious: How do you find Switzerland, as they like to refer to themselves, a startup nation, relative to DataHow?
[00:23:24] Alessandro: Let me be very clear. DataHow exists because of ETH and the unique environment created around ETH, bringing together a huge number of top scientists in many different disciplines. The possibility of being inspired by other ideas is tremendous. But on top of that, ETH is engaged in extensive industrial collaboration. One of ETH's main mandates is supporting the Swiss industry. There is a lot of focus on applied ideas and many initiatives that support these ideas. Additionally, or because of that, Zurich is also a very interesting environment to come up with new ideas with different people. It's a culturally diverse city where more than one-third of the population is foreigners. It's the perfect place to come up with new ideas and develop them with very brilliant people. In this regard, Switzerland is doing a fantastic job of promoting all these ideas. In fact, the number of startups created every year at ETH or, in general, in the Zurich area is incredible, given the size of this environment.
[00:24:57] Ken: Well said. Switzerland always ranks in the top five of the most innovative countries, and ETH, by far, ranks the highest in producing a number of technologies and startups around them. Recently, we saw a rumor that Apple has opened its largest center outside of the US in Zurich, following in the footsteps of Microsoft and Google, who have had a strong presence in Zurich for a while. You're right. Going way back, actually. As we wrap up, I'm curious. How do you maintain your edge as a leader? Do you have any recommendations you'd like to highlight for the audience?
[00:25:40] Alessandro: I always have difficulty perceiving myself as a leader. But in the end, I really like to challenge myself continuously, and frankly speaking, the best way I found to challenge myself was to engage in this startup, DataHow. No more excuses, no more blaming bad bosses, bad colleagues, and so on. It was actually a fantastic way to grow, especially as a person. But in general, I spend a significant amount of time reading and looking at other ideas—the web is an immense source of ideas. In general, I always try to challenge myself from two perspectives. The first is the scientific perspective. I don't know who said this but shifting from the 'know-it-all' attitude to the 'learn-it-all' mindset is important. Never believe that you are the expert who possesses the truth. Always continuously challenge your ideas or perceptions with other ideas, and as we just said, in that regard, Zurich and ETH also offer tremendous possibilities to do that. But I also try to challenge myself a lot on a personal level. That's about how to better engage with my co-workers, be more empathetic, and be more prone to listen to other ideas, which is not always simple, and improve my communication. Ultimately, everything comes down to being very respectful and leading by example. As I mentioned before, there are many tools to improve further. I am reading a lot. Particularly, if I mention very few sources—I'm an eager reader of the Harvard Business Review Journal. They also have a wonderful YouTube channel. But I also follow some people in the English-speaking world, like Simon Sinek, who often challenges my ideas. This may not be so appreciated in the Italian world, but I'm Italian. There is also an interesting person called Marco Montemagno, who helps in continuously challenge yourself at a personal level on how to manage certain processes for a startup. There are a lot of ideas; the important thing is always to remain open to these ideas as much as possible.
[00:28:18] Ken: I certainly would take exception to what you said right up front about not considering yourself a leader because we're investing in you as a leader, and you have been there, done that. You've demonstrated leadership, and that's more important than what one says; it’s about what one does or, more importantly, what one accomplishes. You have a lot to be proud of in terms of your accomplishments, but I like your idea about doing a startup. Some will call the real-world MBA or Master of Business Administration—going out and doing a startup because you learn everything.
[00:28:49] Alessandro: Exactly.
[00:28:51] Ken: Alessandro, thank you for sharing this time and these insights with us today.
[00:28:56] Alessandro: Thanks to you again for hosting me. It's always nice to have this conversation. I hope people will find it interesting and visit our webpage for more information about what we're doing.
[00:29:06]
Ken: Excellent, and we will provide links to the webpage and the resources in the attached podcast when we publish it. This has been Dr. Alessandro Butté, CEO and co-founder of DataHow. Thank you for listening, and please join us for the next episode of our Industrial Impact podcast. We wish you an impactful day. You've been listening to the Momenta Digital Thread podcast series. We hope you've enjoyed the discussion, and as always, we welcome your comments and suggestions. Please check our website at momenta.one for archived versions of podcasts, as well as resources to help with your digital industry journey. Thank you for listening.
[The End]
Connect with Alessandro Butté on LinkedIn!
How to Keep Your Edge
Alexander maintains his edge as a leader through a continuous commitment to personal and professional growth. He embraces challenges, particularly through his involvement in the startup DataHow, where he confronts obstacles head-on, refusing to make excuses or blame others. His approach is rooted in humility, shifting from a 'know-it-all' to a 'learn-it-all' mindset, constantly seeking new ideas and perspectives. He dedicates significant time to reading and exploring various sources, including the Harvard Business Review Journal and insights from thought leaders like Simon Sinek and Marco Montemagno. Alexander prioritizes both scientific and personal development, striving to improve communication, empathy, and leadership skills. By leading by example and remaining open to new ideas, he embodies a culture of respect and continuous learning within his team.
About DataHow AG
DataHow is a Swiss technology company specializing in data analytics and bioprocess modeling that believes that data and smart digital technologies will transform biopharma process operations across process development and manufacturing.
DataHow aims to be a key enabler of the industry’s digital transformation with its’ AI-enabled bioprocess solutions and digital twin technologies. For more information on the company's technology and digital solutions, please visit http://www.datahow.ch.